We live in a data-driven world where data is now more valuable than ever. With the rise of digitalization, these data are being processed to benefit almost every sector. For the smooth functionality of any business institution, systematic research on corresponding data is conducted by an expert. There are different ways through which this research is carried out by experts or data scientists. Data analytics vs Data mining- the two distinct streams of processing data fall under the same umbrella of Business Intelligence.
Depending on these two methods, a specialist can comprehend valuable data and provide insights to the business organization. Consequently, it helps the business grow by making the right decision in future plans and investments. In this way, when a company becomes assured about its performance in today’s competitive market, it gets a lot of scope in satisfying customers and earning better profit.
Here is a guide to Data Analytics and Data Science
Why is It Important to Know the Difference Between Data Analytics and Data Mining?
There’s one similarity between Data analytics vs Data mining- both are parts of Business Intelligence. Other than that, these two have a lot of differences between them. Whereas data mining helps us to understand the pattern of collected information, data analytics goes further to organize that dataset to determine crucial decisions.
The terms Data analytics vs data mining are often used interchangeably, but that is absolutely incorrect. Yes, they are related since data mining is a part of data analytics. The two have different methods and goals.
Data mining, a subset of data analytics, includes tracking patterns, association, classification, detection, etc. Data analytics is a prolonged process that entails statistics, mathematics, and operating systems. Thus the multidisciplinary subject data analytics makes evident use of the findings from data mining.
Therefore, while understanding the minutes of data studies, it is obviously worth understanding the main differences between data analytics and data mining. For a reader who is a novice in this field, both of the topics data analytics vs data mining are individually discussed below for a clear understanding of their differences.
Also Read:
- Data Analyst Skills
- Data Analyst Career
- Data Analytics Scope
- Data Analyst Salary
- Data Analytics Books
What is Data Analytics?
Data Analytics is a broad field of data collection, inspection, and transformation of data to create useful information and discover solutions. This vast field extensively relies on the application of OR (Operations Research), Statistics, and Computer Programming to draw effective conclusions.
With these tools, an analyst gathers valuable information from previous performances; and studies them to establish the most reliable decision. In this era of social media and exclusive usage of search engines, data is spread all over the world.
Honing proper analytical skills, one can dig into this ocean of available information to understand the affinity of the generation.
This results in understanding the customer in every sector, providing them with the best available facilities. Thus a data expert helps you to understand the trends, reveal opportunities, and provide concrete decisions.
Data Analytics comprises many subjects like Machine Learning, Data Science, Applied Statistics, etc. Data Analysis is often confused and misunderstood with Data Analytics.
It should be noted that Data Analysis is one of the subsets of Data Analytics and Data Analysis consists of cleaning, modeling, transforming, and questioning the data.
You may also want to read:
- Data Analysis for Business
- Data Analytics For Finance Professionals
- Data Analytics Tools
- Data Analytics Techniques
- Data Analytics Entry-level Jobs
Process of Data Analytics
Data requirement: The first step in the data analytics process is to decide what type of data you need for your analysis and then proceed to gather them.
Data collection: Once you are convinced of your requirement, you have to look for the sources to avail the data. There are various sources like questionnaires, case studies, direct observation, surveys, etc.
Data cleaning: Before sending the data for analysis, it is important to sort out the necessary data. This will clean up duplicate records or data having any kind of error. This is a mandatory step in organizing useful data.
Data analysis: A number of tools and software for data analysis are available in the market to help you process or analyze the relevant information. Some examples of such tools are – Rapid Miner, Chartio, Redash, Metabase, Excel, R, Looker, etc.
Data interpretation: Once the data are processed using the mentioned tools, it is time to interpret the result and move towards making the most solvent decision using that.
Data visualization: Next is the time for presenting the insights graphically so that it is understandable for everyone. It helps people to read the datasets and compare the results with others. Some popular methods of visualization are – graphs, charts, pie charts, maps, bullet points, etc.
You should know the most important Data Analyst Interview Questions
What is Data Mining?
Data Mining, also referred to as Knowledge Discovery in Data (KDD) is the process of sorting valuable data from vast data sets. With the help of advanced analytics tools and techniques, help businesses predict future patterns and make the most suitable decisions.
Data mining is an important part of Data Science that uses advanced Data Analytics techniques to transform valuable information into creating business planning and strategy. The information it gathers can also be used in Business Intelligence (BI).
In this way, Data Mining not only scrutinizes the historical data but also examines the currently streaming data. Effective Data Mining supports planning business strategies and implementing them. Beginning with marketing, sales, customer support, advertising, manufacturing, etc, Data Mining aids in operating finance and HR.
Even critical cases like fraud detention, security breaches, and risk management, etc are also benefitted by Data Mining. In this way, Data Mining affects not only business but healthcare, government, sports, etc.
Data Mining techniques basically work on two different levels. On one hand, it describes the target dataset. On the other, it predicts the consequences using a machine learning algorithm. In these ways, it surfaces the most important information, filters, and organizes them to create valuable results.
The task of a data scientist is to identify outliers through regression and classification methods. He or she is responsible for extracting necessary information, and collecting and visualizing data for concrete decision-making of the institution.
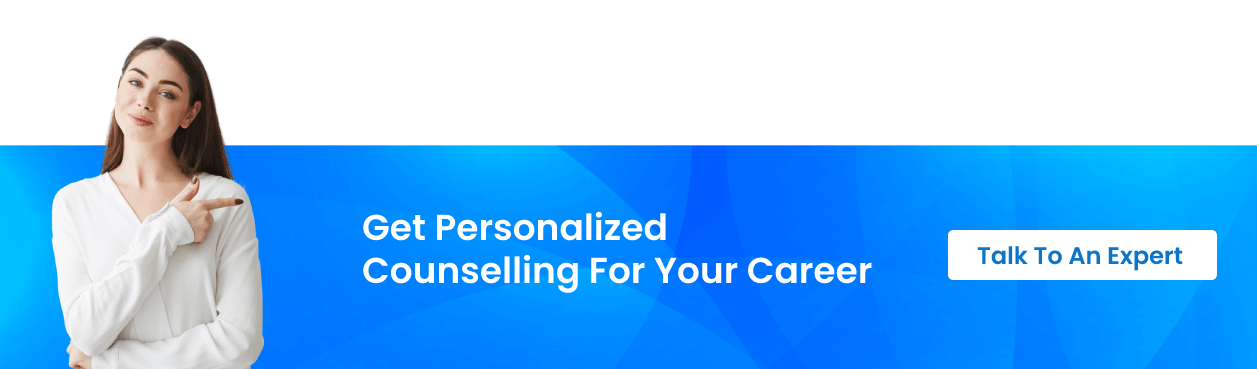
Check here the top-ranked data analytics courses to get a high-paying job:
- Data Analytics Courses in India
- Data Analytics Courses in Mumbai
- Data Analytics Courses in Delhi
- Data Analytics Courses in Kolkata
- Data Analytics Courses in Bangalore
- Data Analytics Courses in Pune
- Data Analytics Courses in Hyderabad
Types of Data That Are to Be Mined:
- Stored data in a database: This is also called a database management system (DBMS). Every data stored in the database is related. Software programs manage these data and help to access them hassle-free. These data are mainly of three types, e.g., shared, distributed, and concurrent data. All these data are stored securely by the software programs.
- Data warehouse: This is a single data storage that gathers data from different sources. The collected data then go through the process of cleaning, integration, loading, and refreshing. After that, the data are stored in a unified plan.
- Transactional data: Every single online transaction has a unique ID. For example, flight booking, online shopping, and even a click on a website – all these transactions are stored in the database and help in the data mining process.
- Others: There are many other varieties of data, like engineering data, multimedia data, data streams, spatial data, etc.
Process of Data Mining
The complete process of data mining follows the below-mentioned four steps-
- Setting of business objectives: This is the foundation of the whole data mining process and is considered the most important one. Business stakeholders should work with the data mining expert to chalk out the main motives and achievable goals of the business. For the data scientist, this can be the hardest part demanding extra time and research to understand the business objectives. After the research, the target should be set by the expert and the same must be sanctioned by the company.
- Preparation of data: Once the goal is marked then it becomes easier to chalk out the plan of data mining. The data scientist will then identify the particular information that will be able to answer the pertinent question to the business. These relevant data are then sorted and cleaned. In this step, all types of noises- e.g., missing values, outliers, and duplicates are removed. If the sorted data contains too many dimensions, then it is again calculated and cut down to only useful predictors, otherwise, too many dimensions may slow down the process of achieving accuracy.
- Model building and pattern mining: Depending on the nature of the collected data, they are modeled with data relationships, such as correlation, sequential pattern, etc. A deep Learning Algorithm may be implemented in this step.
- Evaluation and implementation: The finalized data become ready to be evaluated. If the result meets with the desired output, the dataset is used as a dependable predictor to guide the business with the best knowledge and plan for the future.
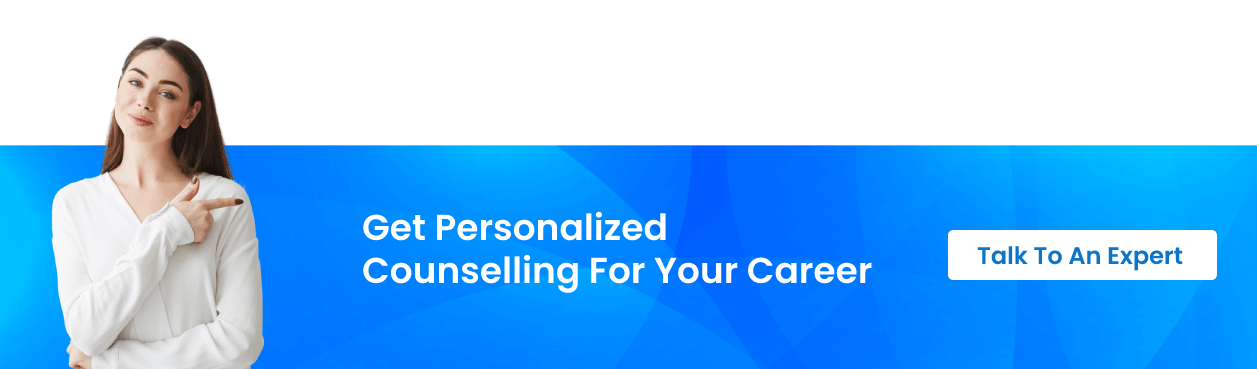
You should check here the best:
- Online Data Analytics Courses
- Data Analytics Courses in Gurgaon
- Data Analytics Courses in Noida
- Data Analytics Courses in Ahmedabad
- Data Analytics Courses in Agra
- Data Analytics Courses in Bhopal
- Data Analytics Courses in Chennai
- Data Analytics Courses in Jaipur
Data Analytics Vs Data Mining- the Key Differences Explained
1 Types:
Data analytics is of four distinct types, they are-
- Descriptive analytics: This uses historical data and uses simple mathematical tools like spreadsheets.
- Predictive analytics: This uses statistical analysis along with Artificial Intelligence to identify possible results.
- Diagnostic analytics: Using machine learning, this digs into historical data to understand patterns and correlations (if any) of data.
- Prescriptive analytics: This uses machine learning and artificial intelligence to help businesses in ultimate business-making. This is the most complex type of data analytics.
The main types of Data mining are-
- Pictorial data mining: This transforms collected heterogeneous data into pictorial presentation. It helps in power plant monitoring, flight control, etc.
- Text mining: This extracts high-quality data from different written texts through a computer. Through statistical pattern learning, data is derived from written texts such as websites, reviews, books, etc.
- Social media mining: More often than not, this data mining is used in traditional or digital marketing. Valuable data is derived from user-generated content in social media and mobile applications to understand and categorize the user.
- Web mining: in this process, useful data is automatically derived from web searches and restored from the World Wide Web. The result is used in e-commerce, content analysis, health care services, etc.
- Audio and video mining: Multimedia or audio-visual is very accessible nowadays. From this video, huge data sets are extracted and then applied in the fields of security, entertainment, education, and medicine.
2 Tools:
There is no single tool that can address all data analytics or data mining tasks. If you can find one tool that satisfies most of your needs, there must be a secondary tool for some other tasks left.
- The tools essential for data analytics are-
Microsoft Excel, Python, R, Jupiter Notebook, Apache Spark, SAS, Tableau, KNIME, etc.
- The tools for data mining are-
Orange, SAS, DataMelt, Rattle, Rapid Miner, etc.
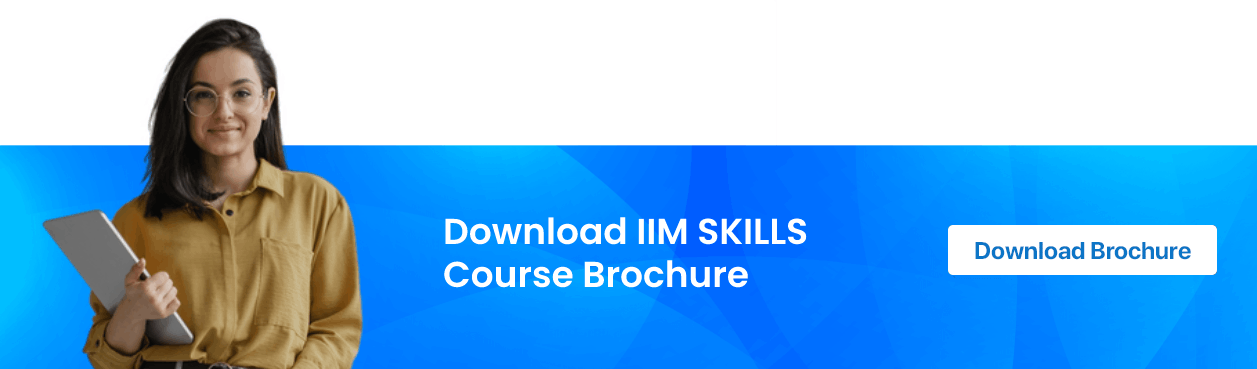
Learn more about:
- Data Analytics Using Power Bi
- Data Analytics vs Machine Learning
- Data Analytics in Project Management
- Data Analytics and Artificial Intelligence
- Data Analytics With Python
3 Method:
Let us now highlight the different methods in both data analytics vs data mining-
Some of the most used methods for data analytics are-
- Cluster Analytics- This is the process of finding objects of similar groups to form clusters. This algorithm is based on machine- learning and works on unlabelled data. Clustering can be done in different ways, such as – density-based, constraint-based, distribution-based, centroid-based, etc
- Cohort Analysis- This is behavioral analytics that categorizes data in a data set into cohorts or groups that share the same traits or behavior. By analyzing, the cohorts can understand well about their attitudes and choices.
- Regression Analysis- This method is used to realize the relationship between two or more variables. The result is presented in a graph through independent and dependent variables.
- Segmentation Analysis- In this method, users or products are divided into different groups to deliver customized advertising, brand positioning, etc.
- Time series Analysis- This method is useful for non-stationary data, that is, data that fluctuates over time. Industries like retail and finance make use of this method.
Data Mining methods are-
- Association: In this method of data mining, the co-occurrence of associated items is found in a collection. For example, when a shopper puts different items in a basket, it is possible to recommend some other associated items.
- Classification: Through classification, we assign a class label to each item in a dataset. Classification is of two types- binary classification and multi-class classification.
- Clustering Analysis: It is the process of grouping similar objects into one cluster. For, at first, the partition of data is made and then the label of the group is assigned according to their distinguishing features.
- Prediction: By processing input datasets, the numerical output is generated through an algorithm. Unlike classification, this numerical output does not contain any label.
- Decision trees: This structure contains root nodes, branches, and leaf nodes. The internal node denotes tests, the branch denotes the outcome of the test, and the leaf node denotes a class label.
4 Subject-matter expertise:
In data mining the following skills are quintessential-
- Knowledge of Linux:
- The idea about a programming language:
- Knowledge of essential tools:
For data analytics, the below-mentioned prerequisites are needed-
- Learning of data visualization tools:
- Knowledge of programming language:
- Probability:
- Econometrics:
- Programming language:
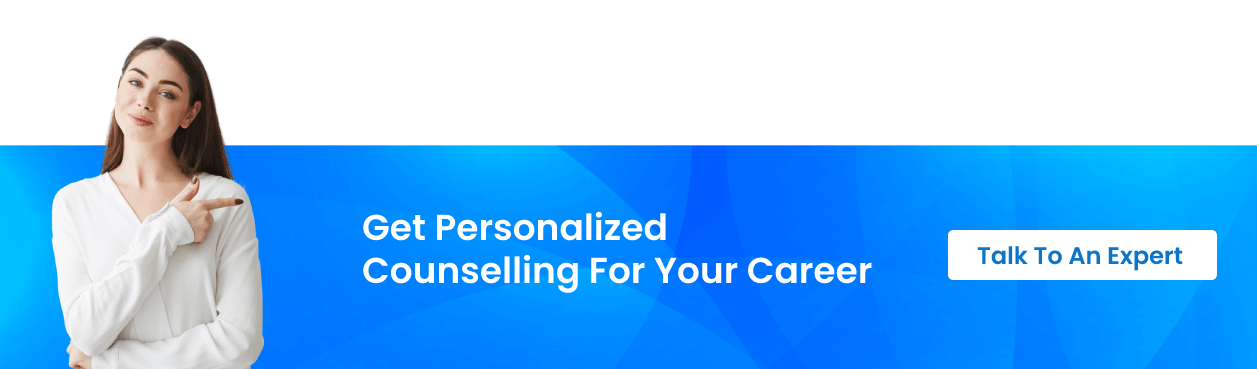
5 Hypothesis- testing:
A hypothesis is a supposition made with limited evidence and needs further investigation to be approved.
- Data analytics heavily depends on the hypothesis that gives information to be tested. For that matter, it even tests on the results derived from data mining.
- The data mining expert does not need to conduct a hypothesis. He comprises the collected data or discoveries in a mathematical or statistical.
6 Skills Required:
The different skills required for data analytics vs data mining are discussed below.
- Data analytics skills:
- Probability and statistics: These two are the founding pillars of data analytics. Using these skills, an analyst estimates value for further research. The statistical approach is mainly based on probability.
- Data visualization: In data analytics, it is very important to visualize the outcome through charts, graphs, etc. This visual representation demonstrates the complicated result in a simpler way so that all the stakeholders understand and can take decisions relying on them. One common visualizing tool is Tableau.
- Programming language: There are some very useful programming languages to make the analyst’s task smoother. Beginning with Excel, he can hone his knowledge of programming languages like R, Python, etc.
- Econometrics: It is a branch of economics that deals with statistical and mathematical models. With the knowledge of econometrics, the analyst can foresee future trends and make better predictions.
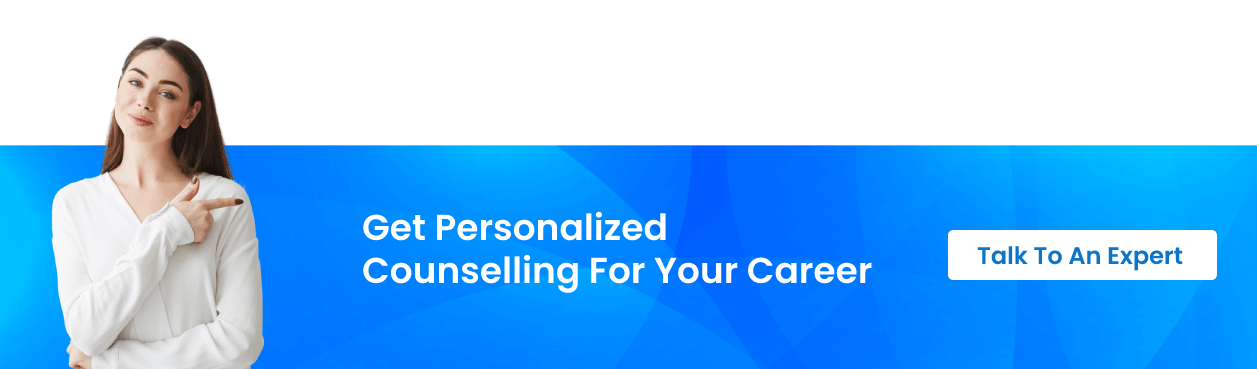
- Data Mining skills:
§ Programming language: A set of programming languages used for data mining. They are- JAVA, Matlab, SQL, Python, R, etc. Depending on the dataset one working with, a particular program is selected. However, the most common of them are Python and R.
- Data processing frameworks: These frameworks extract insights from large datasets. Some of the most implemented frameworks are Hadoop, Spark, etc.
- Operating system: Linux is the most popular OS for the data mining process.
- Database knowledge: To process the large dataset, one must have knowledge of Relational databases (SQL, Oracle) and non-relational databases (Document, MongoDB, Cassandra, etc.)
7 Size of the Team:
- Data analytics is a lengthy process that usually requires a group of people. This team will ask questions, draw patterns of responses and reach a conclusion. Although machine learning, Artificial Intelligence, etc. are used in this process, the lengthy process requires human involvement.
- Data mining can be conducted by a single person also. With the right technological skills and knowledge of software, he will write down the data findings.
Frequently Asked Questions on Data Analytics vs Data Mining
1 Which is more in demand – Data Analytics vs Data Mining?
Data analytics help in making hypotheses whereas data mining makes data useable. So, both of them have different fields of application. As data scientists are currently ruling the world with new-age science and technology, there is a dearth of experts in both. None of the above-discussed streams are conventional stereotypical subjects to have huge competition and lots of competitors. So, one has only to hone their capability to be hired by big companies.
2 In brief, what is the main difference between Data Analytics vs Data Mining?
Data mining is a step in the complicated process of data analytics. This step focuses on scientific and systematic methods of data collection and then, deriving insights from those data. Data analytics goes on further by using those data to create hypotheses and build models on that.
3 Name four main models that are used in Data Analytics.
The four main models for data analytics are –
- Descriptive
2 Diagnostic
3 Predictive
4 prescriptive
4 Write about two useful models used in Data Mining.
1 Predictive model / Statistical Regression: As the title says, through this model, data are converted to form predictions about the future performances of the company.
2 Descriptive model: In this model of data mining, data are described in a readable format. It extracts from the stored data and creates reports by monitoring them.
5 Data Analytics vs Data Mining- write some applications of each.
- Application of data analytics:
Data analysts can help strengthen security by supplying valuable data on crimes to police. Geographical and historical data analysis of a particular crime-driven area helps in locating such spots and eventually necessary action can be taken.
- Application of data mining:
Data mining applications can help in business revenue by investing capital in an efficient way. The sale of a particular item at a particular time of the day can be researched using data mining techniques. The production and availability of that particular item will help in better revenue for the business.
Conclusion: Data Analytics vs Data Mining
With the wide usage of computers research on data has taken a leap. Although the two terms data analytics vs data mining – are often used interchangeably, the above description points out their distinction. On one hand, these differ in implementation. On the other, the experts in the two fields require different skills. With the sheer power of computers, experts are playing with data to explore more in this field. Between data analytics vs data mining, whichever side you may choose, the above details enlighten you to appreciate the importance of the other. The data-driven 21st century needs mindsets with a knack for coding to make a revolution. So, this was the guide to data analytics vs data mining.