The ability to make an informed decision on the basis of the analysis of data has become an ultimate game changer for every technologically driven business. The abundance of data that we observe around us drives today’s environment. In recent years, it has become inevitable for businesses to consider the importance of data. Hence, many large-scale industries are demanding resources skilled in data analytics and machine learning. If you are unsure of which career will foster future growth, you should think about enrolling in one of these courses. Let’s comprehend data analytics Vs. machine learning in the most researched manner in this article.
Data Analytics Vs. Machine Learning:
Let’s start with understanding all the facets and subcomponents of data analytics Vs. machine learning. We can commence our proceedings by understanding its terms.
Here is a guide to Data Analytics and Data Science
What is Data Analytics?
Data analytics can also be referred to as “data analysis.” The process of gathering unorganized data, visualizing it, and arranging it in a way that makes sense to the business and aids in making decisions is known as data analytics. Data analytics refers to the process of analyzing collected data to identify trends and make judgments about the information it contains.
The process of data analytics is largely carried out by the use of specialized systems of hardware and software. All large-scale companies depend on data to improve their products and services. But these data are nothing when they are not statistically sorted into algorithms. That’s where the role of the data analyst comes into existence.
Data analysts will carry out a data-driven approach to help businesses make crucial decisions related to their products and services. Data and algorithm-driven businesses are going to be the next pioneers and business leaders. Companies can use data analytics to improve processes, increase revenue, boost customer service, make informed decisions about production and sales, increase employee productivity, and catalyze tailored customer approaches.
Companies that initiate data analytics are more likely to react quickly to current market trends and can obtain an advantage over rival companies. People who excel as data analysts are creative problem-solvers who pay close attention to detail, comprehend statistics and quickly pick up new technological skills. A career as a data analyst is an option if you enjoy trying new things.
Also Read:
- Data Analyst Skills
- Data Analyst Career
- Data Analytics Scope
- Data Analyst Salary
- Data Analytics Books
What is Machine Learning?
Machine learning is a subset of artificial intelligence that helps any software program make decisions based on previous algorithms. Its main objective is to automate data collection from historical sources and arrange it into patterns that support decision-making.
Using statistical and predictive analysis, traditional machine-learning techniques scour the available data for patterns and hidden information. Modern machine learning, in contrast, uses supervised, unsupervised, and reinforced techniques to investigate pattern recognition and advance computational learning theory in artificial intelligence.
One of the best instances of machine learning at work is on Facebook, YouTube, Netflix, and many more. Facebook’s machine learning system examines the behavior of the user and recommends articles, shows, and news according to the interests of the user. It makes use of the user’s prior platform searches and bases its recommendations on the user’s preferences.
In the same manner, when Amazon recommends products and when YouTube and Netflix suggest shows, nothing but machine learning is at work. Data analytics focuses on using data to find insights, whereas machine learning focuses on creating and perfecting algorithms so that they can operate independently of human input.
You may also want to read:
- Data Analysis for Business
- Data Analytics For Finance Professionals
- Data Analytics Tools
- Data Analytics Techniques
- Data Analytics Entry-level Jobs
Let’s Discuss Data Analytics vs. Machine Learning Comparison
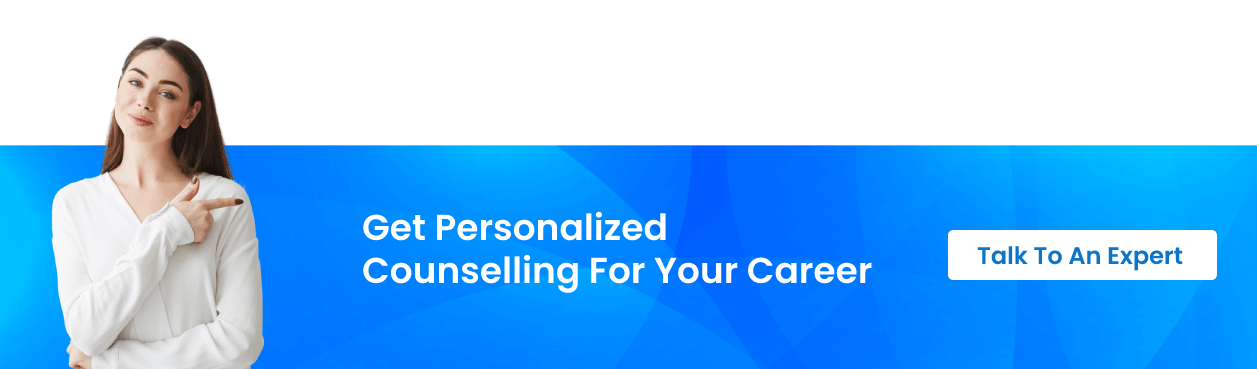
Data Analytics Vs. Machine Learning: Keyskills
The necessary skillset to develop to make a career in the field of data analytics are,
- You need to learn a programming language like R or Python. The rich libraries of these languages will assist you in working with data easily.
- In order to access and use databases, you must become familiar with a structured query language.
- You must possess strong presentation skills to present your findings in front of customers, stakeholders, or businessmen.
- You should have knowledge of mathematics and statistics.
- You must have the skill of collecting data and understanding its behavior.
The necessary skill to develop a career in the field of machine learning is,
- You must have a deep knowledge of mathematics and statistics.
- You must know basic software programming.
- You need to familiarize yourself with machine learning libraries and packages.
- Problem-solving and analytical thinking are a must for machine learning.
- Good communication and teamwork skills.
You should know the most important Data Analyst Interview Questions
Data Analytics vs. Machine Learning: Roles and Responsibilities
Roles and responsibilities in the field of data analytics
If you are willing to enter the field of data analytics, you will spend your valuable time collecting and analyzing data. A data analyst is essential for problem forecasting or offering a worthwhile solution to an existing issue.
His presence in an organization has varying meanings for various business organizations. However, in general, a data analyst’s job responsibilities include the following.
- Data collection from internal and external sources is the first responsibility of a data analyst. Data can be collected through surveys, transactional tracking of customers, gathering online behavioral data, and keeping watch on social media. There are a number of ways in which research can be conducted, and they vary from business to business.
- The data collected through different sources might contain duplicate and repeated data. To avoid any skewed data, data analysts clean the data.
- All the dispersed and random data must be fed into a tabular or graphical form to simplify the analysis process.
- The quality of the data plays a very important role in enhancing the productivity of the organization. Data analysts, therefore, verify the relevance of the information for the company.
- The data and information are the assets of the business. Hence, securing the data and information has also become one of the key responsibilities of the data analyst.
- A data analyst must possess strong presentation skills to represent his findings in a meaningful way. The reports prepared by the data analyst must help in monitoring the profitability of the company.
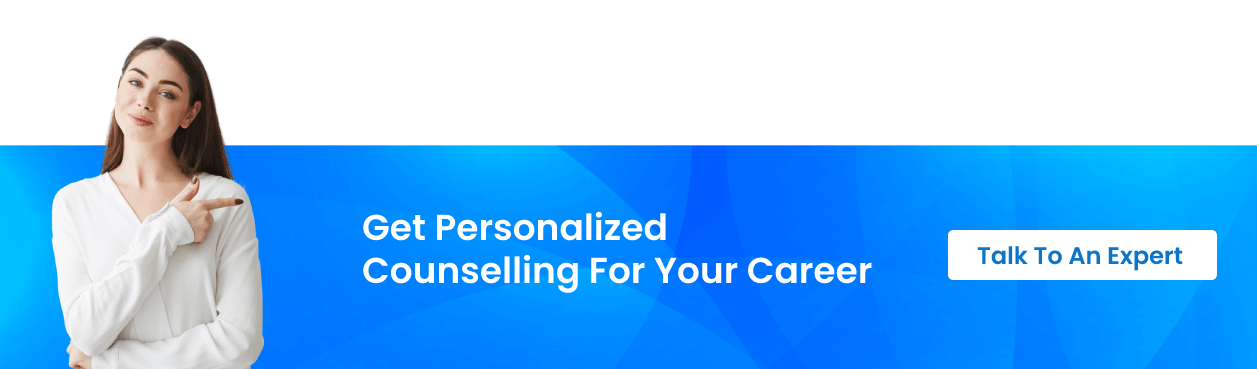
Roles and responsibilities of a machine learning engineer
- Investigating and putting into practice the models developed by data analysts would be your primary responsibility if you decided to become a machine learning engineer.
- You will be entitled to build machine learning algorithms.
- Due to the fact that outdated versions will not be useful, you will need to update the models and machine learning systems in accordance with the specifications.
- You must possess strong communication and interrogation skills to become a machine learning engineer. As a part of your job, you will need to communicate with data analysts, data scientists, and other administrative staff to develop the algorithms of machine learning.
- On the basis of the data gathered from different sources and the models created by the data analyst, you will generate machine learning algorithms that are capable of learning and formulating predictions.
- A machine learning engineer must possess strong programming skills in Python and R. However, he is not required to develop any API. He is only expected to be familiar with the software companies’ developed API packages and libraries. He is anticipated to possess integration skills.
Check here the top-ranked data analytics courses to get a high-paying job:
- Data Analytics Courses in India
- Data Analytics Courses in Mumbai
- Data Analytics Courses in Delhi
- Data Analytics Courses in Kolkata
- Data Analytics Courses in Bangalore
- Data Analytics Courses in Pune
- Data Analytics Courses in Hyderabad
Data Analytics Vs. Machine Learning: Thriving Industries.
Today, there are many industries that depend almost entirely on data analysts to gather data and machine learning engineers to automate decision-making. Let’s broaden your perspective on the various sectors that employ data analysts and utilize machine learning.
Data Analyst Expert: Thriving Industries
The retail and wholesale sectors use data analytics to refine pricing strategies and investigate the causes of erratic shifts in demand. They also anticipate competitors’ actions in the market with the use of data analytics. Banking and Financial institutions will get insights from the data gathered in curating customer-specific service instead of standardizing banking programs for all customers.
The investment sector in the banking industry monitors the financial market by making use of data analytics techniques. For example, the stock exchange market makes use of data analytics to detect unethical trade practices. The healthcare industry is one such sector where making a quick decision is inevitable.
In this situation, data analytics are crucial to making a quick decision that yields successful results. Data analytics provides well-informed recommendations to decision-makers based on the patient’s clinical data. It increases the effectiveness of the healthcare industry.
The weather forecasting model enables data analysts to prevent natural disasters like floods. Short-range forecasting, medium-range forecasting, and long-range forecasting are the three types of forecasts that are made possible with the use of data analysis.
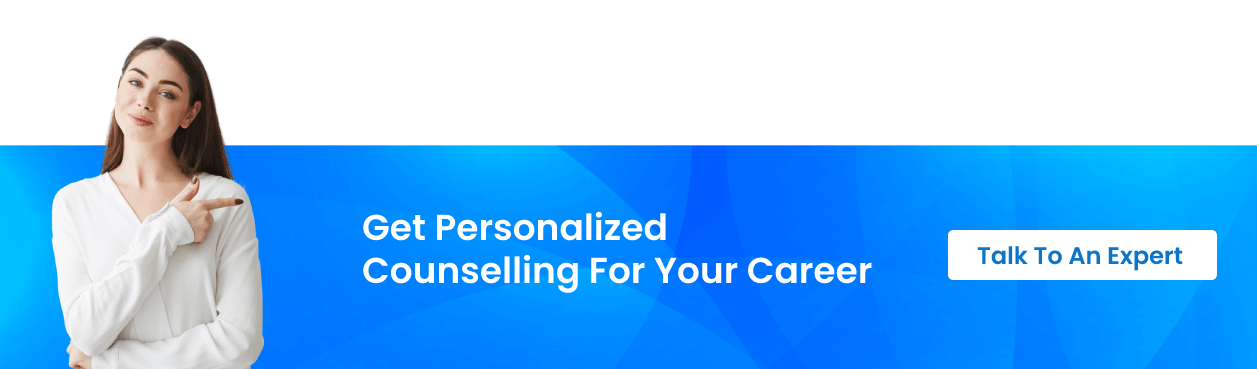
Machine Learning Engineers: Thriving Industries
Machine learning is helpful in regulating different processes of software development. The software development industry is booming at an alarming rate, and so is the need for machine learning engineers. Machine learning eliminates the need for human intervention in making decisions and driving actions.
Machine learning algorithms are very powerful at discerning patterns from the data fed into the system. Machine learning can deal with a large amount of data very easily when compared to humans, and marketing is one such sector that is overflowing with data. With the use of machine learning, it becomes easy to find patterns in users’ behavior and build advertising campaigns accordingly.
When estimating production that can be in line with demand, machine learning offers a workable solution for manufacturing industries. This helps in reducing costs and easily detecting errors. Supply chain management and asset tracking are added advantages of employing machine learning in manufacturing industries.
Machine learning plays a vital role in the cybersecurity of an organization. Machine learning can identify patterns and suggest preventative steps that should be taken against cyberattacks. Machine learning is a powerful technology that even delivers real-time solutions to respond to active attacks.
Machine learning offers umpteen benefits to the finance sector. Machine learning can analyze millions of data points in a short period of time and offer meaningful insights into trading patterns. Machine learning paves the way for safe and secure digital transactions.
Machine learning is benefiting the healthcare sector in several ways. The wearable device is the result of machine learning and can monitor heartbeat, steps, calories burned, blood pressure, and many more on the list, which aids doctors in treating patients in real time.
You should check here the best:
- Online Data Analytics Courses
- Data Analytics Courses in Gurgaon
- Data Analytics Courses in Noida
- Data Analytics Courses in Ahmedabad
- Data Analytics Courses in Agra
- Data Analytics Courses in Bhopal
- Data Analytics Courses in Chennai
- Data Analytics Courses in Jaipur
Data Analytics Vs. Machine Learning: Tools Used.
There are many tools used for offering services, such as machine learning engineers and data analysts. However, no one tool can suffice to solve different business cases. Even if none of the tools seem to be a great fit for you, using a combination of them can be the best option because most of them work well together.
Here are some common tools used in data analytics vs. machine learning.
Tools used by the data analyst are,
- Microsoft Excel
All organizations use Microsoft Excel to sort data in a meaningful manner on a regular basis. You can use Microsoft Excel as a tool to improve your data insights. It is one of the most widely used tools for data analytics and offers users options like sharing workbooks, working on the most recent version for real-time collaboration, and uploading data.
Python is frequently chosen by data scientists and analysts to evaluate data. Because of its simple syntax, ease of learning, and extensive library of tools for numerical computation, graphics, and data visualization, Python has grown in popularity.
- R
R programming is an open-source language that is widely used for statistical computing and graphics. Data analysts use this tool to identify patterns and build practical models. The programming language contains a large library that offers interactive visuals and facilitates easy analysis of data visualization and representation.
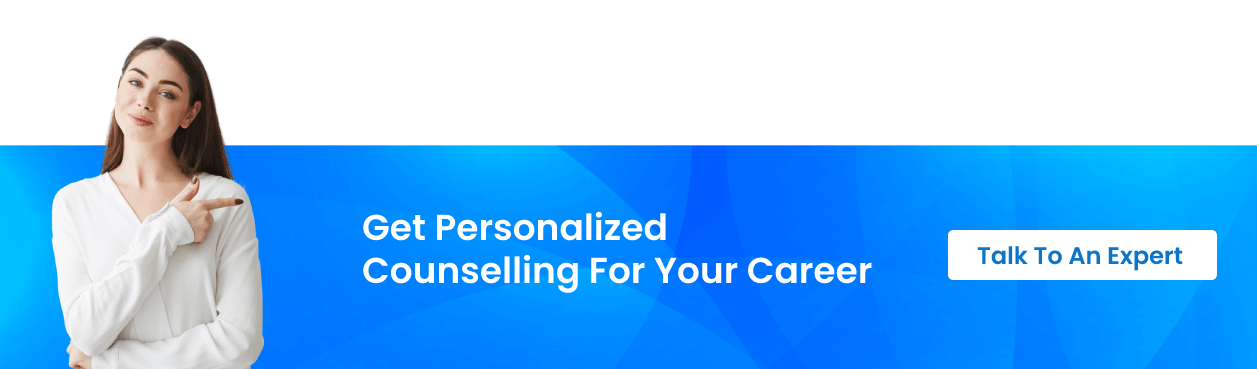
- Jupyter Notebook
Jupyter Notebook is a code editor that is widely used by data analysts these days because of its user-friendly environment. Jupyter Notebook can be hosted on your local computer which makes it ideal for data analysis and visualization. It supports numerous languages, including Python and R.
- Apache Spark
Apache Spark is used to process Big Data Workloads. It is an open-source distributed processing engine. Its in-memory caching and improved query execution make it possible to run quick analytical queries against any size of data.
- SAS
SAS stands for statistical Analytics Software. This tool is used by large-scale organizations because of its high reliability in computing complex statistical operations.
- Microsoft Power BI
Microsoft Power BI is a comprehensive data analytics tool that aids in comparing past and present data that will affect the future of an organization. It offers comprehensive guidance on the percentage change in revenue, customer experience, and success of the organization.
- Tableau
Tableau is a data analytics platform that allows one to prepare, analyze, and share the insights collected through big data. Tableau allows working on the live data set, which allows one to spend more time on data analysis rather than data wrangling.
- KNIME
KNIME stands for Konstanz Information Miner, which is a free and open-source data analytics, reporting, and integration platform built for working on a GUI-based workflow. One of the key features of this tool is that it does not require any prior programming knowledge to implement.
Learn more about:
- Data Analytics vs Data Mining
- Data Analytics Trends
- Data Analytics vs Machine Learning
- Data Analytics in Project Management
- Data Analytics and Artificial Intelligence
- Data Analytics With Python
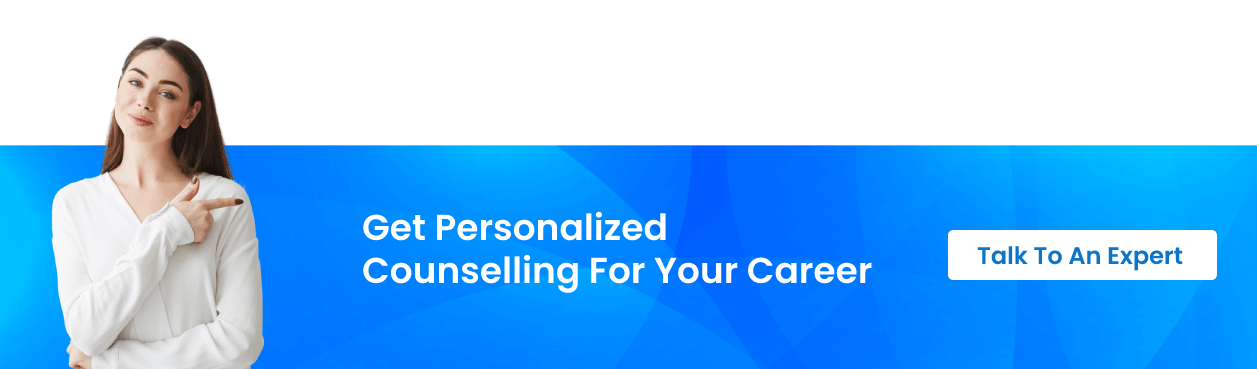
Tools used by machine learning engineers are,
- TensorFlow
TensorFlow is an open-source platform for machine learning. Due to its extensive libraries, which make numerical competition simple, it is very well-liked among machine learning experts, especially for large-scale machine learning and deep learning projects.
- PyTorch
PyTorch is an open-source machine learning program. Python and Torch Library are the cornerstones of PyTorch. It is considered one of the most popular platforms for deep learning research. The framework is designed to hasten the transition from research simulation to implementation.
- PyTorch Lightning
PyTorch Lightning is easy to install and can be easily integrated with other machine-learning tools. Its key feature includes its ability to print alerts when a developer makes any mistake in code.
- Scikit Learn
Scikit Learn is free software in Python with a bunch of algorithms. It is more about creating and building models, so one must have basic knowledge of Python programming, supervised and unsupervised models, and other commonly used libraries.
- Catalyst
Catalyst is an additional PyTorch framework created especially for deep learning applications. It supports research and handles engineering responsibilities like code reuse and reproducibility, which enables quick experimentation.
- XGBoost
XGBoost is mainly used for two reasons. One is the speed of execution, which is crucial when working with large data sets. And the other is model performance, which makes your models stand out from other models.
- Light GBM
The power of Light GBM cannot be underestimated because of its ability to place continuous values into discrete bins, which leads to faster and more efficient usage of memory. It is capable of handling large-scale data because of its faster training speed and support for parallel and GPU learning.
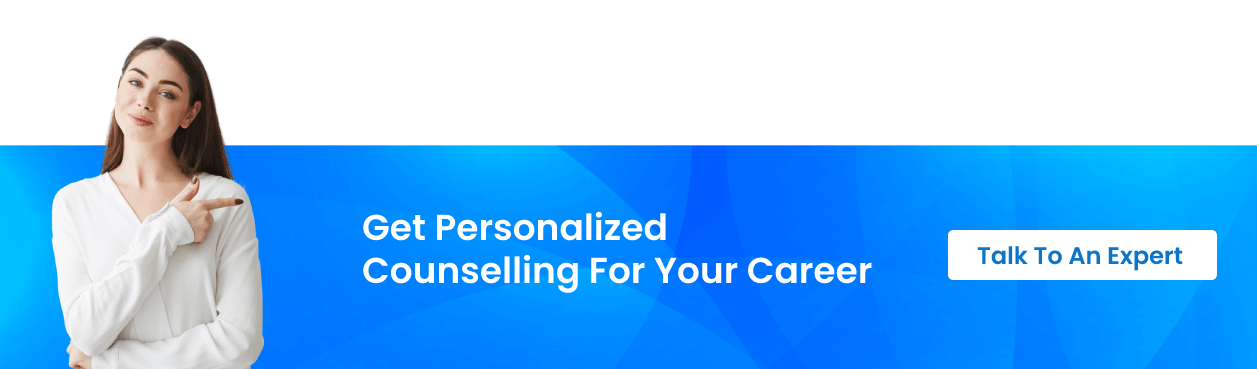
- CatBoost
Gradient-boosted decision trees are the foundation of CatBoost. A series of decision trees are built sequentially during training. Training is faster, and models are more accurate, with the use of the CatBoost tool.
- Fast.ai
The advantage of Fast.ai is that deep learning should be usable across several operating systems, languages, and small datasets. It was created to eliminate a significant amount of unnecessary engineering labor.
- PyTorch Ignite
PyTorch Ignite provides a simple user interface to increase research capabilities and save unnecessary coding. PyTorch Ignite has a standard version and works well in an ecosystem of integration to assist machine learning.
Data Analytics Vs. Machine Learning: FAQs
Q1. Data Analytics Vs. Machine Learning, Which is the better choice as a career?
Ans1. Both learning data analytics and machine learning offer a great career foundation in their own specific ways. Being a differently promising career, knowing each in detail is truly imperative. A career in data analytics revolves around collecting and understanding the data, finding patterns, and observing the underlying patterns to draw meaningful inferences. Experience in using various data analysis tools and methods is required to mold a career in data analytics. In machine learning, computer programs are developed to make decisions on the basis of preprogrammed algorithms. Both are great career options, and choosing one largely depends on one’s area of interest.
Q2. What is the future of a data analyst?
Ans2. As many businesses are moving towards a data-driven process of decision-making, the role of a business analyst is getting notified to a large extent. Once you gain experience as a data analyst, you can take on the roles of project manager, functional consultant, IT PMO, pre-sales consultant, etc.
Q3. Which computer language do I need to learn to enter the field of machine learning?
Ans3. The most prominent computer languages that you can master to enter the field of machine learning are Python, C++, Java, Javascript, and R.
Q4. Are data analytics Vs. machine learning both different terms?
Ans4. Yes, they are both totally different terms. Data analytics is about using various analytical tools and techniques to derive valuable insights from the collected data. Data analytics and machine learning are two key methods used by a data scientist to derive meaningful information from the data and become part of a crucial decision-making team. In machine learning, computer programs are created to use algorithms that are built into them to make decisions and learn on their own. Data analytics, on the other hand, is the process of drawing conclusions from the data.
Q5. What are the job profiles in data analytics?
Ans5. The job profiles of data analytics include Data Scientist, Data Architect, Data Analyst, Statistician, and Database Administrator.
Conclusion About Data Analytics Vs. Machine Learning:
I hope that by this point, you are much more aware of the distinctions between data analytics Vs. machine learning. While the former focuses on extracting patterns and insights from the data, the latter uses the data to train and test algorithms. However, because they are both technological fields, they heavily rely on one another. Please share your thoughts in the comment box on this article data analytics Vs. machine learning.