Several factors are responsible for building a profitable business. In a society powered by technology, these factors can be collected as data, organized and analyzed to study target audience demographics & preferences, predict markets, and make informed decisions for building a profitable business. Experts have identified several data analysis techniques that are at a business’ disposal for increasing its customer base and accordingly, profits. In this article, we have listed the top data analytics techniques and also a few software that help businesses analyze with ease.
Table of Content
- What is Data Analysis?
- Importance of Data Analysis
- Types of Data Analysis
- Process of Data Analysis
- Top Data Analytics Techniques
- Data Analytics Tools
What is Data Analysis?
In simple words, Data Analysis is the practice of inferring useful information from raw data collected by personnel from different sources. Data Analysis in any field is useful for building, maintaining, and growing a thriving business in the dynamic economic and social scenario.
Data Analysis can be used for gathering information regarding the inner workings of an organization, the audience preferences, or shifting market trends.
All organisations need to invest in data analysis for efficient decision-making regarding all internal and external matters covering everything from audience demographics and market trends to production line and personnel performance checks.
Also Read:
- Data Analyst Skills
- Data Analyst Career
- Data Analytics Jobs
- Data Analyst Salary
- Data Analytics Books
Importance of Data Analysis
A business is based on the principle of trading certain products or services in exchange for financial profit or fulfilling non-profit causes. To enable trade, businesses need to put their best foot forward at every turn to convert interest in their product or service into a sale.
Data Analysis gives the businesses a quantifiable and qualitative measurement of their operational parameters. This further initiates a uniform comparison with standards and lets the businesses initiate improvement in areas to achieve their goals.
Here is a guide to Data Analytics and Data Science
Different Data Analytics Techniques Are Required for a Business to:
Improve Organisational Decisions
Data Analysis reduces human errors. The process of data analysis enables business owners to make informed decisions based on collected, processed and analyzed data so that guesswork and subsequent mistakes can be minimized for the organization.
Several data analytics techniques can be used to infer information from raw data to implement efficient decision-making at every level in the organization.
Enable Cost Efficiency
The economies of a business are affected by multiple fluctuating factors. While these factors are recognized, their fluctuations need to be studied and predicted to ensure that the business’s finances always have an increasing graph. Data Analysis in business helps them identify and restructure budgets, costs, and expected profit margins for the business’ continued operations.
Increase Sales Conversions
A business’s primary objective is to sell. Data Analysis lets the management identify the needs, wants, and attractive aesthetics and results required by the audience in a product or service. Taking care of the audience preferences as identified through various data analytics techniques can be the deciding parameter for a business’ sales conversions.
Improve Customer Relations
The present media scenario enables two-way communication between a business and its audience. This raises the business’s responsibility toward its audience’s expectations regarding the products and services. Data analysis helps businesses organize data into categories and also identify and evaluate dynamic customer interests and concerns. This is part of the last step of efficient management for any business.
You may also want to read:
- Data Analysis for Business
- Data Analytics For Finance Professionals
- Data Analytics Tools
- Data Analytics Scope
- Data Analytics Trends
Types of Data Analysis
Data Analysis can be broadly classified into Qualitative and Quantitative analysis. The other types of analysis can be treated as subcategories of the above. To succeed in business, organizations need to identify their needs and employ a balance of qualitative and quantitative data analysis in their evaluation.
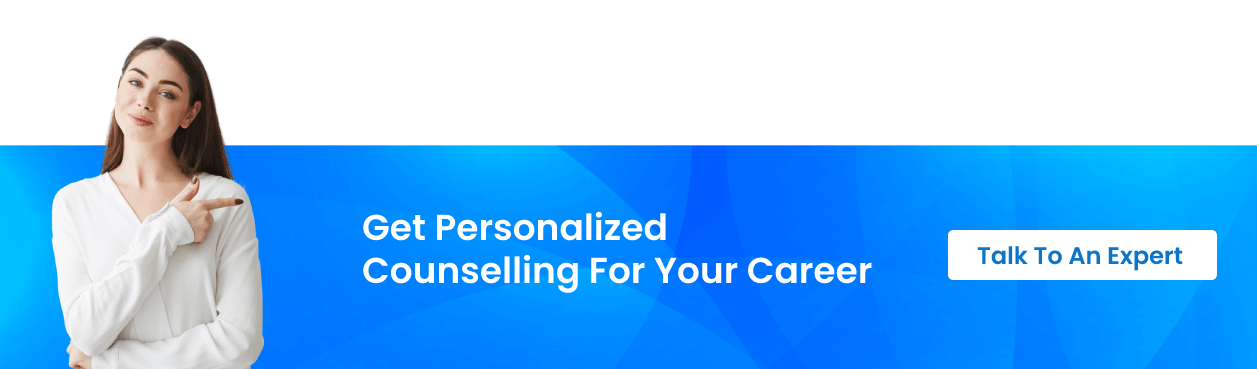
Qualitative Analysis
The qualitative data analysis does not give a numerical result of the processed data. Simply put, a qualitative analysis is meant to answer the why, what, and how of a problem. For example, the data collected through open-ended questions for a customer feedback interview will give the analysts a general opinion regarding the product, service, and image of the organization in the customer’s eyes.
Quantitative Analysis
As the name speaks, a quantitative analysis seeks to provide measurable or quantifiable results to the data. A quantitative analysis can give you numerical results for multiple problems like:
- Sales Conversion in a quarter or area or through a medium
- Performance appraisals of employees
- A comparative study with a competitor’s sales
- Evaluating the working conditions for personnel and machinery and many more.
Text Analysis
Also known as text mining and information extraction, text analysis involves the compression and conversion of complicated or lengthy heterogeneous content into easy-to-read data pieces to make them readable by machines. Text Analysis can be complicated due to the several languages spoken today. In such cases, machines display all results that have logical validity.
You should know the most important Data Analyst Interview Questions
Statistical Analysis
Statistical Analysis is the interpretation of processed data with the help of statistical operations and visualizations like pie charts, bar graphs, etc Statistical Analysis lets the business give information at a glance making it easier to understand, justify, and act upon.
Diagnostic Analysis
Diagnostic Analysis is a more in-depth form of statistical analysis that seeks to answer particular questions through data discovery and processing. It seeks to identify the root cause of any particular problem. Diagnostic Analysis is performed to solve a problem. Thus, it may also involve data collection from external sources if it correlates with the problem in question and may even be a cause for it.
Predictive Analysis
Predictive Analysis is performed in preparation for the future. It can be involved in all future concepts including market trends and patterns study, increasing operational and managerial efficiency, preparing for and reducing risk factors and even improving present and future marketing campaigns.
Learn more about:
- Data Analytics vs Data Mining
- Data Analytics Using Power Bi
- Data Analytics vs Machine Learning
- Data Analytics in Project Management
- Data Analytics and Artificial Intelligence
- Data Analytics With Python
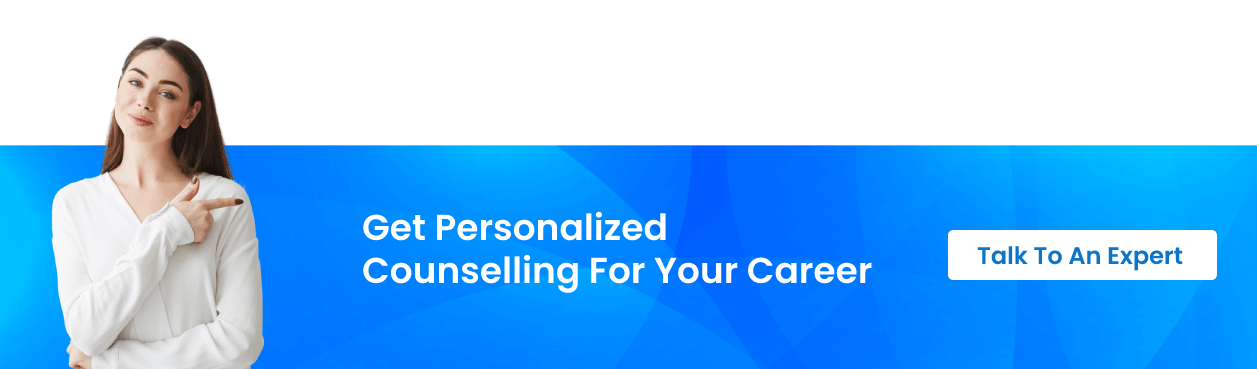
Process of Data Analysis
To be of use, Data needs to be collected, classified, analyzed, and interpreted into an understandable concise format to enable its use for different business functions.
The Process of Data Analysis Includes the Following Steps:
Define the Problem
Before beginning the analysis process, the problem that needs to be solved and the objectives to be fulfilled need to be set by the data analysts of the organization. Without having specific goals, the data cannot be converted to useful results.
Collection of Data
After setting the objectives, the organization can collect raw data from internal and external sources that will enable them to infer positive or negative results. The data may be collected from primary or secondary sources.
Data Cleaning and Classification
Even after the collection, the data is not usable without being organized and compact. The collected data needs to be sorted through, organized, classified, and compiled into understandable pieces of information.
Exploration and Analysis of Data
By using various analysis techniques, the data is analyzed and visualized to predict the results from the data. By using these techniques, the analysts can infer the relationships between the factors and that with the subject.
Interpret the Results
After the analysis, all that is left is to interpret the result according to the set objectives and determine whether the results meet the objectives or not.
Looking to opt for the data analytics courses? Here are the best:
- Data Analytics Courses in India
- Data Analytics Courses in Mumbai
- Data Analytics Courses in Delhi
- Data Analytics Courses in Kolkata
- Data Analytics Courses in Bangalore
- Data Analytics Courses in Pune
- Data Analytics Courses in Hyderabad
10 Best Data Analytics Techniques
Data analysis can be performed through various data analytics techniques based on mathematics, statistics, artificial intelligence, and machine learning. Some of the widely used data analytics techniques are mentioned below:
1. Regression Analysis
Regression Analysis is a statistical analysis technique that seeks to define the strength and properties of a dependent factor with one or more independent factors of the data. A Regression Analysis lets you study the impact of the factors on each other. Regression Analysis can be differentiated according to the properties of their variables.
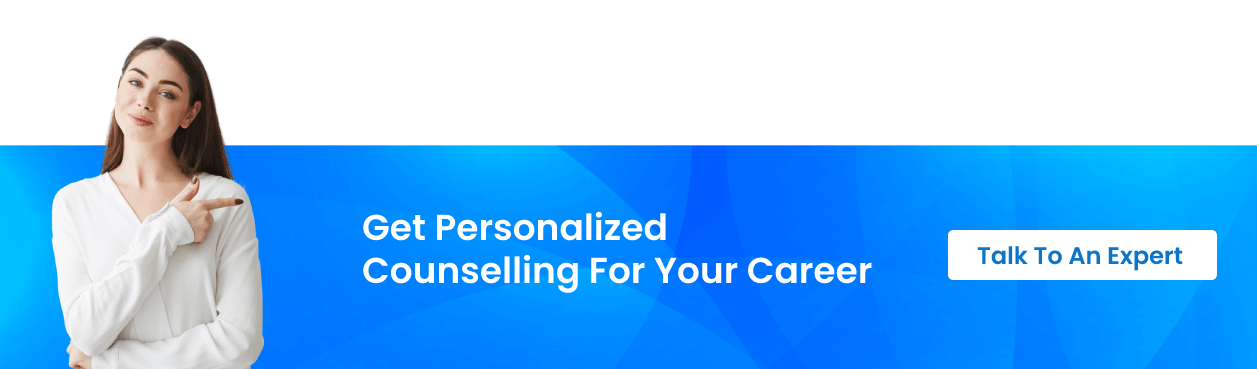
When the variables may take any value within a range, they become Continuous Dependent Variables and may use linear or nonlinear regression analysis, the former of which can be further divided into the following:
- Fitted Line Plots
- Stepwise Regression and Best Subsets Regression
- Ridge Regression
- Lasso Regression
- Partial Least Square (PLS) Regression
When the variable gives qualitative results through exclusively classified observations, they are known as a Categorical Variable which can be analyzed through the following regression:
- Binary Logistic Regression
- Ordinal Logistic Regression
- Nominal Logistic Regression
Variables may also take small exact countable values which are then termed as Count Dependent Variables. The data analytics techniques used to analyze these variables are:
- Poisson Regression
- Negative Binomial Regression
- Zero-inflated Models
2. Factor Analysis
Factor Analysis, also known as Dimension Reduction Analysis, is the technique that lets us reduce the number of factors into smaller observable units with the understanding that several variables are correlated and have one concept according to which they can be grouped under a single entity through covariance.
This technique is especially helpful as it lets analysts sort through a bombardment of individual data and move ahead with analysis with the use of grouped data which highly relates to our objectives.
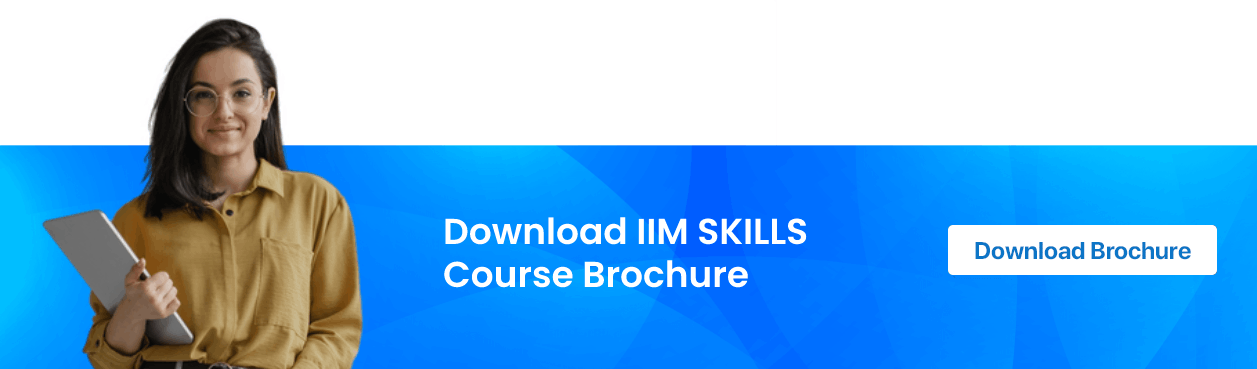
3. Monte Carlo Simulation
The Monte Carlo Simulation or Monte Carlo Method is one of the most popular data analytics Techniques for risk analysis. This technique is a computerized analysis that seeks to predict the multiple outcomes of a situation and also the probability of each outcome happening.
This data analysis technique enables organizations, be it business, finance, engineering, or even online gaming.
Monte Carlo Simulations have three components: input variables, output variables, and the mathematical model. The latter of the three is the most important component to produce the output variables or possible results. The following steps must be followed when conducting data analysis through Monte Carlo Simulation:
- Formulate an equation from the available data according to which a mathematical model will be built.
- Determine the values that should be included to form the model and group them into sets.
- Set up and operate the Monte Carlo Simulation software like GoldSim, Excel, or Amazon Web Services.
- Analyze the results to solve the problem.
You should check here the best:
- Online Data Analytics Courses
- Data Analytics Courses in Gurgaon
- Data Analytics Courses in Noida
- Data Analytics Courses in Ahmedabad
- Data Analytics Courses in Agra
- Data Analytics Courses in Bhopal
- Data Analytics Courses in Chennai
- Data Analytics Courses in Jaipur
4. Cohort Analysis
Cohort simply means a group. Thus, cohort analysis is the process of performing the data analysis on people who have been grouped based on a particular trait. This technique enables analysts to identify patterns in the data that may be specific to a cohort with similar preferences, financial brackets, or even the time from which they have been using a business’ product or service.
Cohort Analysis is one of the data analytics techniques that enables businesses to tailor their services for specific cohorts as identified under the analysis giving their consumers superior before, during, and after-sales services.
Love working with numbers? Check out these articles below:
- Data Science Courses in Noida
- Data Science Courses in Faridabad
- Data Science Courses in Indore
- Data Science Courses in Jaipur
- Data Science Courses in Kota
5. Cluster Analysis
Cluster Analysis is an exploratory data analysis technique that seeks to apply set analysis algorithms on naturally grouped data. It is an exploratory method that works on data that appears exclusive of other clusters and similar to data within the cluster.
It lets the analysts recognize the underlying structures in the data but does not go into the depth of what causes the occurrence of these structures within a dataset.
Cluster Analysis is a technique that prepares the data for further analysis. The several algorithms that may be applied in this technique are:
- Partition based Algorithms
- Hierarchical Algorithms
- Density-based Algorithms
- Grid-based Algorithms
- Model-based Algorithms
- Constraint-based Algorithms
- Outlier Analysis Algorithms
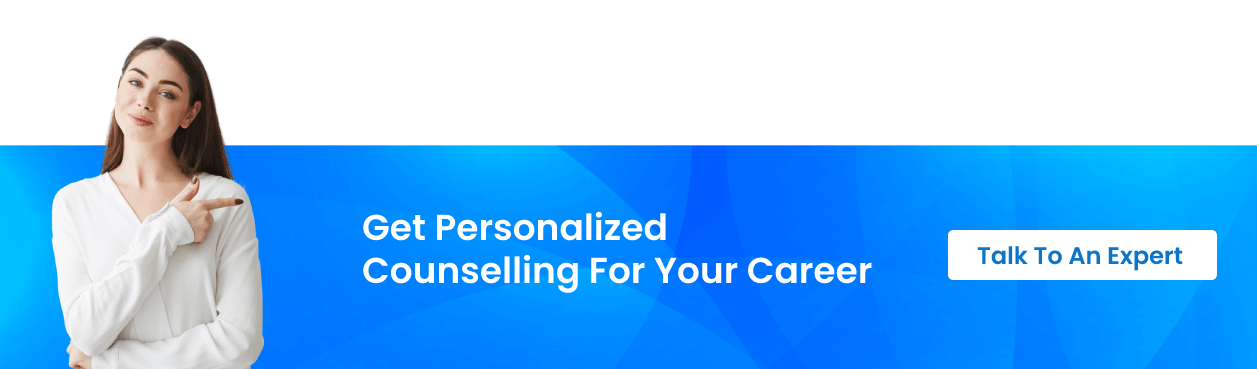
Recommended Read for Statistics Enthusiasts
- Data Science Courses in Pune
- Data Science Courses in Gujarat
- Data Science Courses in Ranchi
- Data Science Courses in Raipur
- Data Science Courses in Surat
- Data Science Courses in Trichy
- Data Science Courses in Trivandrum
6. Time Series Analysis
This data analytics technique seeks to analyze data at different points in time. This analysis seeks to establish the changes in resulting data with the same variables at different points in time.
The time series analysis seeks to establish time patterns in the data like trends, seasonality, and other cyclic patterns. The simplest example of the usage of the marketing campaign for seasonal products like air conditioners, inners, and heaters.
The time series analysis can be conducted through various models which can be broadly classified into:
- Autoregressive Models
- Integrated Models
- Moving Average Models
Recommended Reads:
7. Sentiment Analysis
Research has proven that the audience makes maximum decisions through an emotional quotient. If any action of a business knowingly or unknowingly harms the sentiments of the audience, it could result in heavy losses for the business.
In contrast, the audience may also ignore slight problems if they feel a sentimental connection with the business brand. With this in mind, businesses conduct sentiment data analysis to predict whether the delivered message will have a positive, negative, or neutral emotional quotient.
These data analytics techniques seek to improve brand reputation by making favorable operational decisions. While the approaches to sentiment analysis can be divided into Rule-based, Machine Learning, and Hybrid; sentiment analysis is of different types:
- Fine-grained scoring
- Aspect based
- Intent-based
- Emotional Detection
It is important to note that due to the nature of the analysis, sentiment analysis also has the disadvantage of the variable nature of sentiments. As sentiments cannot be numerically judged, the sentiment data can be subject to sarcasm, negation, and emotional range that may not be limited to a specific emotion.
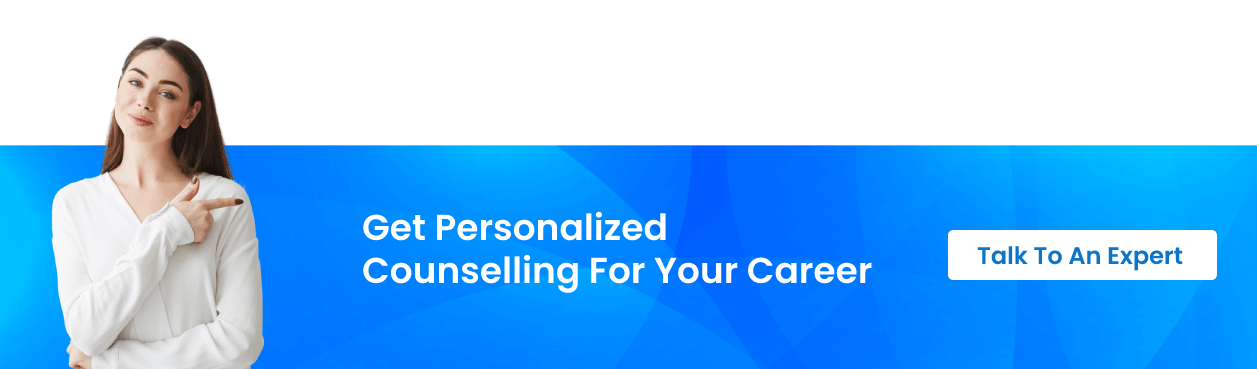
8. Fuzzy Logic
Data Analytics also accepts that market scenarios cannot be subject to exact mathematical values as they are based on human actions, capabilities, and thought processes. The Fuzzy Logic analytics technique thus, studies the data in a way that allows for several outcomes for the positive fulfilment of objectives.
This data analytics accepts the presence of several ‘true’ results instead of an absolute solution as is acceptable for businesses. The Fuzzy Logic is a technique that shares several similarities with other data analytics techniques like Machine Learning and Neural Networks. The components of Fuzzy Logic analysis are:
- Fuzzification
- Fuzzy Knowledge base
- Inference Method
- Defuzzification
9. Machine Learning
Machine Learning is a data analytic technique that seeks to teach a computer to process data in a certain way. It can be treated as a subset of artificial intelligence that uses algorithms to process Big Data repeatedly to build workable models for data analysis according to situations.
Widely used for data mining, machine learning is on the rise due to its property of reducing human effort. Machine Learning includes the use of algorithms like Neural Networks and Decision Trees that are data analytics techniques in their own right but also include:
- Association and Sequence Discovery
- Expectation Maximisation
- Principal Component Analysis
- Nearest Neighbour Mapping
- Singular Value Decomposition
10. Artificial Neural Networks
Simply put, this technique uses the principle of analyzing data like a brain would. As many experts define the human brain as a computer, the artificial neural networks work as a brain would. This is a deep learning analysis that changes its technique structure according to the data.
The data is entered in layers, known as node layers, and works on a model that is built on connecting nodes that are activated if a certain threshold is crossed.
The Artificial Neural Networks technique is capable of processing and analyzing simple or complex data at unprecedented speed and accuracy that is difficult at the same pace by humans.
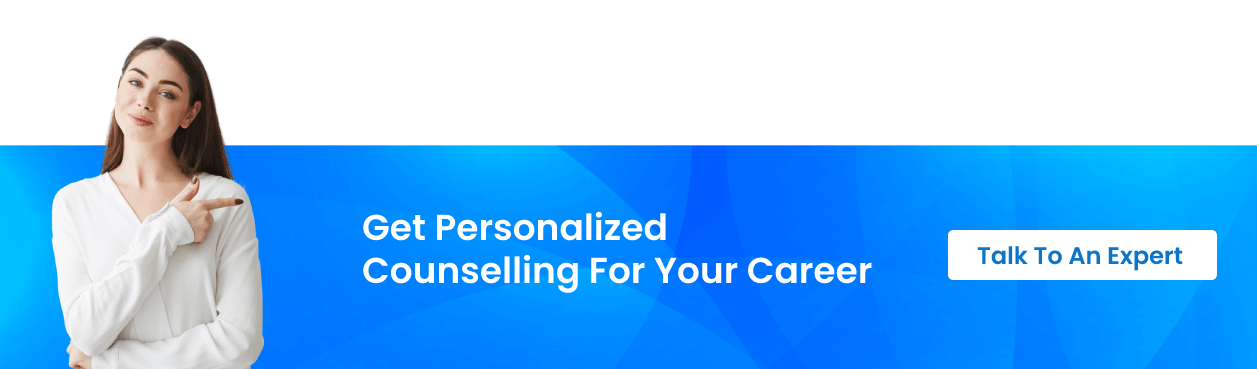
Check here for the other best courses:
- Data Analytics Courses in Delhi
- Data Analytics Courses in Mumbai
- Data Analytics Courses in Gurgaon
- Data Analytics Courses in Bangalore
Data Analytics Tools
Data Analysis is an important practice for businesses. According to their needs, businesses may choose from several tools that simplify the process of data analysis.
Microsoft Power BI
Microsoft’s Power BI is a tool that uses a ‘drag and drop’ feature that enables you to get speedy insights for the input variables in record time. The ease of using the software is one of the most important factors that increases its popularity. Another factor is the availability of the features for a minimal price.
Microsoft Excel
Microsoft Excel is a well-known software that enables superior data organization. Along with organization, it has multiple functions that may be performed to analyze data. Businesses may even install plugins with the software to get a versatile data analysis tool.
Tableau
Tableau is a visual data analytic platform that was developed through contributions by Stanford. The purpose of the platform is to derive data-driven results that may uncover trends and patterns that may be missed through simple analysis. Tableau also has a superior data cleaning facility that users can test through the trial offered by the company.
SAS
SAS analysis software is capable of analyzing data for various mediums including new-age social media. It is especially useful as it can access data from multiple sources that may not have been possible from other software or tools. Its ability to customer profiling is unique to the platform.
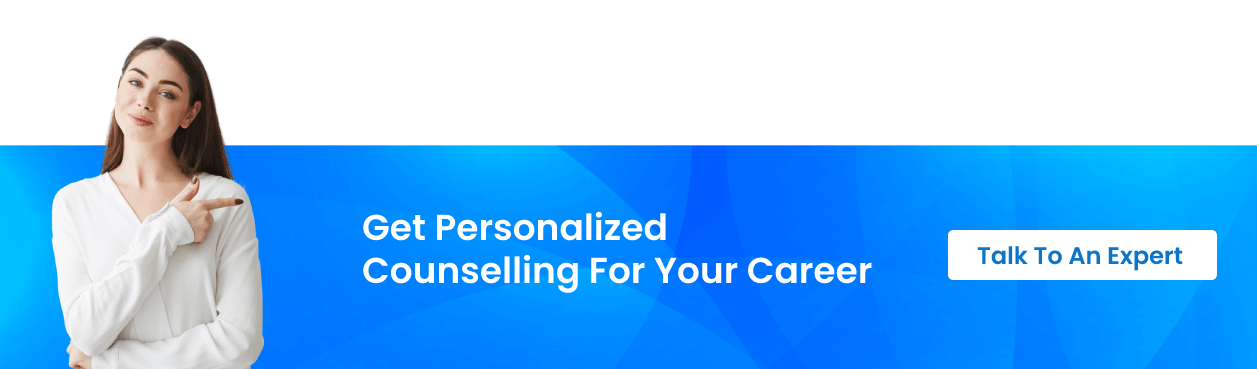
Apache Spark
Apache Spark is an open-source analysis software that conducts real-time processing of data and makes its interface available in 4 widely used programming languages. Apache Spark is easy to use and also offers the Apache Spark and Scala Certification Training Course to train professionals in using the software.
Frequently Asked Questions
Here are the FAQs about the data analytics techniques.
1. What is Data?
In common meaning, Data is the collected form of information that has been translated into a form that is measurable and capable of further study and analysis for solving problems and gaining solutions. It is used to present information in a way that is bereft of repetitions and extra variables. It is classified into qualitative and quantitative data.
2. What are the available jobs in Data Analysis?
As the competition rises in the business sector, business organizations are developing data analytics teams to gain an edge over their competitors in the market. In the Indian scenario, Naukri.com the recruitment website lists over 4000 listings for data analysts. The field has entry-level designations of Junior Data Analyst, Junior Data Scientist, and Associate Data Analyst while senior professionals may be promoted to Business Analysts, Data Engineers, Data Scientists, Marketing Analysts, and more with an average pay range of INR 4 lakhs to INR 10 lakhs.
3. How to choose data analytic tools to perform data analytics techniques?
Having multiple data analytics tools at your disposal can clutter a business’s data analysis process. Choosing an analyst tool becomes an analysis itself with many factors to consider, the basic of which include the scale of operations, budget, and security of the business but also considers interface specifics like the tool’s speed of processing the data, understanding of the programming languages, scale of data handling and more. While they may use the free tools available with ease, businesses should consult an expert before investing in a data analytic tool according to their needs.
4. What is the difference between Data Analytics and Data Science?
In simpler terms, Data Science is a higher-level function than Data Analysis. A data scientist is more involved with operations specific to the business and is responsible for inferring insights for a business specific to it after the general analysis has been conducted. Data Analysis is a branch of data science that seeks to perform specific operations to produce specific insights into data.
Conclusion
Data Analysis is a procedure that is rooted in the continued development of a business organization and the growth of business image among clients and competitors. As technology progresses and the audience evolves as an intelligent entity, the successful analysis and interpretation of their preferences in products, services, and communications is a guaranteed way of building, maintaining, and growing a loyal customer base. By using the best data analytics techniques, businesses can equip themselves with future predictions and past knowledge to tackle the dynamic market to emerge as a successful entity.