We live in the 21st century. The world around us is constantly changing and data has become unquestionably the most valuable asset. Data is essentially the information that is stored or transmitted electronically. Everything we see on computers and the web from YouTube to Google searches to emails to Excel sheets is a form of data. It is essential for everyone be it individuals, businesses, or governments and the demand for data is rising exponentially. This article is a guide to data analytics in project management. Must read to gain in-depth knowledge about it.
Most of the Business decisions are taken based on data and we just can’t get enough of it. It is widely believed that future wars will be fought over data and with the help of data, but raw data is like raw food, it’s not of much use unless we perform some actions on it.
In itself is just a set of numbers or words and to make sense of it we need to analyze and evaluate it so that raw data becomes useful and actionable information and this is done with the help of data analytics.
Here is a guide to Data Analytics and Data Science
What is Data Analytics?
Data analytics is the process of collecting, evaluating, processing, and analyzing data to find correlations, trends, and insights that can be translated into informed decisions.
It is important for organizations to understand the effectiveness of their past decisions in terms of measurable or quantifiable indicators as well as help them to understand consumer behavior in a better manner. It essentially provides scientific backing behind a decision. Data Analytics usually involves the following steps:
Define the Question-Defining the questions means deciding on what exactly one wants to know. It is very important as it gives direction to the whole process of data analytics.
Data Collection– Data collection involves gathering data from online platforms, online surveys, Excel sheets, and databases. It is necessary to make sure that data is relevant in relation to the questions the organization aims to explore with the help of data analysis as the kind of data used will have a direct bearing on the conclusions.
Cleaning the Data-Cleaning of data is making data accurate and free from errors. This process involves looking for missing values, removing inconsistent values, getting rid of duplicate values, identifying superfluous data, and deleting it. The Cleaning of data is essential if want to get credible results.
Analyse Data– This step involves the use of various techniques and statistical tools to explore and understand the data in a more cohesive manner.
Data Visualization– After analyzing the data, the data is presented in visual forms such as pie charts, bar graphs, and dashboards. Data visualization helps in sharing large amounts of complex data into easily interpretable information.
Data Interpretation and Decision Making-Data Interpretation involves reviewing the data. It helps in understanding patterns, trends, and inter-relation between different variables so that concrete conclusions can be drawn from the data and effective decisions can be taken.
Also Read:
- Data Analyst Skills
- Data Analyst Career
- Data Analytics Scope
- Data Analyst Salary
- Data Analytics Books
What is Project Management?
Before we understand the use of data analytics in project management, let us first understand what is meant by project management. Project management is a process through which the project goals are achieved with the available resources.
It involves detailed planning and effective allocation of company resources with the goal of solving the problem within the given deadline. Project Management is done in the following steps.
Project Definition– It defines the scope of the project and determines its objectives and limitations. The project definition should meet the expectations of all the stakeholders such as the client, the company, and the employees.
Project Planning– it involves a wide range of activities such as budgeting, scheduling, organizing, and distributing work. The roles of different individuals are defined and milestones are set.
Resource Management– It involves allocating and managing resources to achieve objectives set in the project definition. It is a very important part of project management as the resources are scarce most of the time and there is a need to make optimum utilization of resources to meet project management goals.
Risk Management– Risk Management deals with figuring out and analyzing the potential risks associated with the project and also providing a roadmap to deal with such risks. There are different types of risks associated with a project such as financial risks, operational risks, or market risks. Risk management helps in developing strategies that will reduce as well as hedge the risks the project is exposed to.
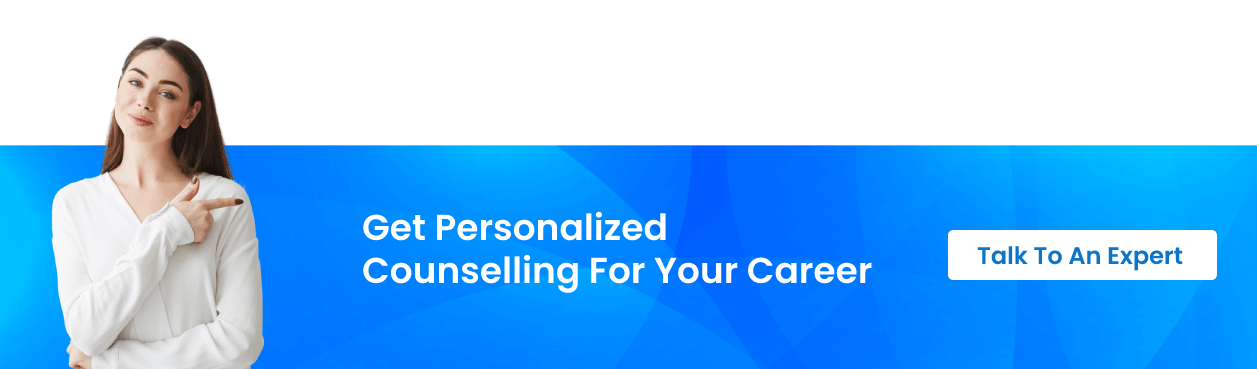
You should know the most important Data Analyst Interview Questions
Project Execution– Project execution is the most important step in the project management process. It involves rolling out the project. It involves coordinating the team, monitoring progress, and coming up with solutions to meet the project objectives. Effective planning can help in smooth project execution.
Communication and Stakeholder Management– Communication is the key to the successful implementation of any project. It is necessary to have an unhindered and clear flow of information to team members so that they understand their roles and execute the project properly. Clients should also get timely information about the progress of the project and milestones achieved so that they can suggest changes if they require any.
Quality Management– Quality Management deals with maintaining standards in terms of project delivery. The quality could be assessed in the form of certain predefined criteria and regular quality checks to make sure that the quality of the project is not compromised.
Project Closure-It is associated with the completion of the project which usually aligns with achieving objectives that are set out in the project definition. It also involves the evaluation of problems that were faced during the implementation of the project.
You may also want to read:
- Data Analysis for Business
- Data Analytics For Finance Professionals
- Data Analytics Tools
- Data Analytics Techniques
- Data Analytics Entry-level Jobs
Data Analytics in Project Management
The project Management process involves interpreting and analyzing data for different objectives such as risk management, project execution, and Quality Management.
Data Analytics can be used to break down complex data with the help of charts and graphs and uncover new patterns that can help in understanding the behavior of different variables thus data analytics becomes the bedrock of project management. Data Analytics is used in project management in the following ways:
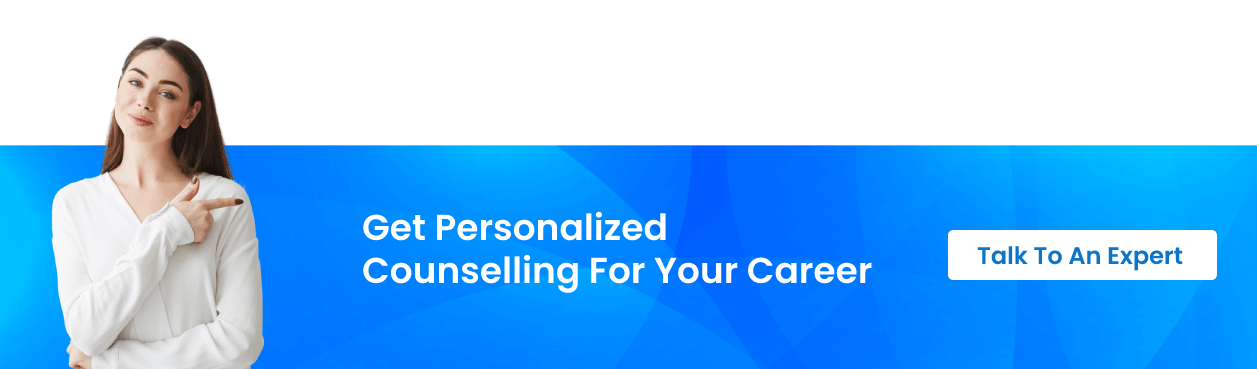
Visualization-Data Analytics is of great use to project managers as it helps in simplifying complex and large amounts of data into easily understandable visual representations. It becomes easier to identify patterns with the help of visual representation as compared to identifying them on data tables.
Different types of visual representation tools are used for different types of data for example, Bar charts are used for discrete values such as expenditure by customers whereas line charts are usually used for showing data over a period of time.
Performance Monitoring-Data Analytics plays a key role in enabling the stakeholders to monitor the performance of the project. Data analytics helps in figuring out the key variables that are essential in meeting the goals set in terms of Key Performance Indicators (KPIs) so the performance can be measured in a quantifiable manner and corrective action can be taken in the areas the performance is lagging.
Risk Management– Data Analytics has a special role when it comes to assessing and mitigating risks. Data analytics can be used for risk identification, risk assessment, risk mitigation, and risk monitoring by analyzing large sets of historical data and identifying patterns that are leading to an increase in risks.
For example, In the financial industry Income data of past customers could be analyzed to identify the customers who could default on their loans.
Check here the top-ranked data analytics courses to get a high-paying job:
- Data Analytics Courses in India
- Data Analytics Courses in Mumbai
- Data Analytics Courses in Delhi
- Data Analytics Courses in Kolkata
- Data Analytics Courses in Bangalore
- Data Analytics Courses in Pune
- Data Analytics Courses in Hyderabad
Resource Optimization– Data Analytics plays a key part in resource optimization as resource optimization is one of the most important goals for any project manager. Data Analytics can be used to find out the areas where resources are not fully utilized and any bottlenecks that are occurring such data can be used for making more efficient policies.
It can also be used for predicting demand so that inventory and working capital are managed accordingly. For example, Bar charts are used for representing discrete values whereas.
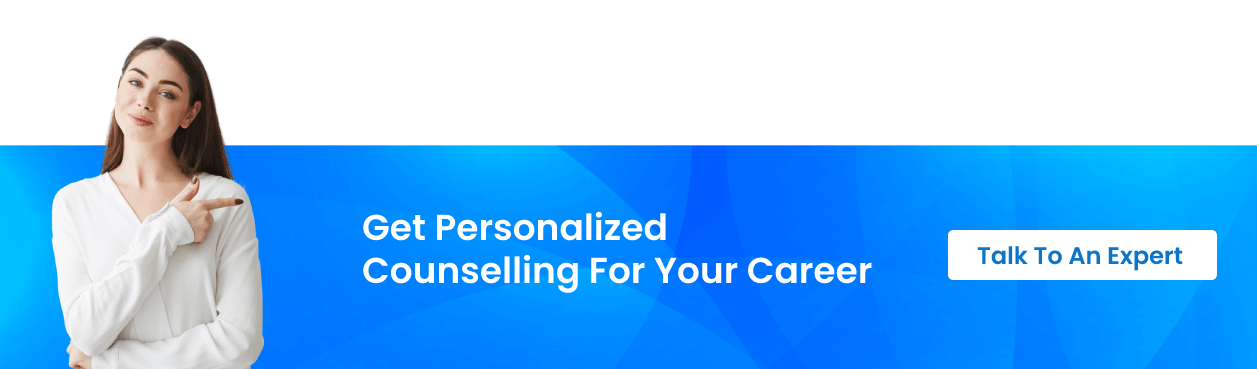
Forecasting and Planning-Forecasting is one area where data analytics plays a key role with the use of tools such as Time series analysis. Time series analysis to find out patterns in historical data about sales or inventory and thus make informed decisions. Machine learning algorithms could be used to identify patterns in data and identifying such patterns will provide vital information in planning.
Tracking Progress– Data analytics is very important in tracking the progress of the project by using the data analytics to identify the main milestones and Key performance indicators (KPIs), Continuous tracking of these milestones will give insights that could be vital inputs in taking corrective action if required.
Stakeholder Management– For any type of project there are different kinds of stakeholders such as customers/clients, the company’s management, and the team members.
Data Analytics makes it easier for each one of them to track timely developments in the project and take a logical course of action. For Example, Data regarding the performance of team members could be analyzed and tasks could be given according to their efficiency in different areas.
Prescriptive Analysis-Descriptive analysis uses past data to identify recurring patterns and general trends whereas predictive analysis is used to predict future performance with the help of data.
Prescriptive analysis uses both descriptive and predictive analysis and goes beyond just finding trends and aims to suggest the best course of action for the project manager thus making the decision-making process a lot less complex.
Managing Project Portfolios-Data Analytics can be very useful for project managers when they are dealing with multiple projects at the same time. Data analytics will make it easier for the managers to identify the projects that are lagging behind in deliverables so a project manager can divert more resources and time to such projects analyzing data from the different projects will also help in identifying trends and the kinds of project that require any special attention and resources.
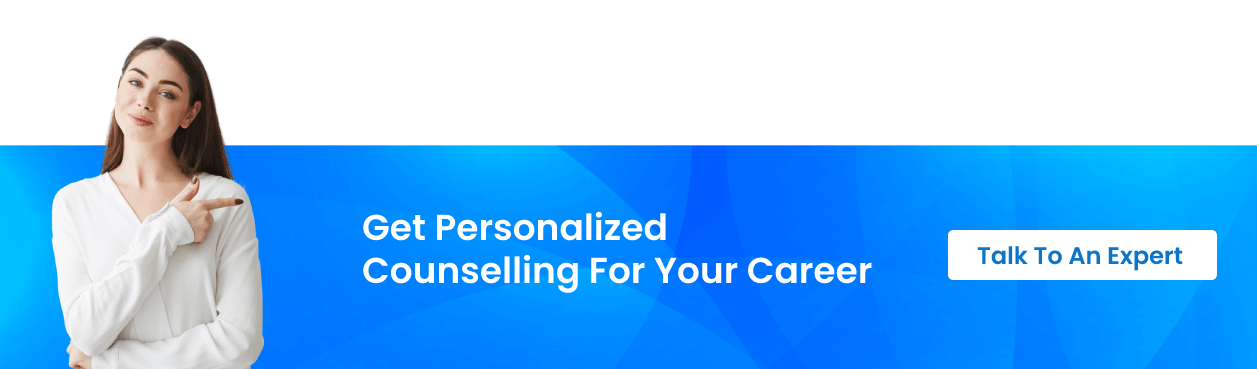
Project Integration across various disciplines– Data Analytics could guide project managers with the help of Project integration as most of the modern-day projects are not department-specific and require the expertise of various departments. By integrating data from various departments, a project manager can get a more holistic view of the project and make more efficient decisions.
You should check here the best:
- Online Data Analytics Courses
- Data Analytics Courses in Gurgaon
- Data Analytics Courses in Noida
- Data Analytics Courses in Ahmedabad
- Data Analytics Courses in Agra
- Data Analytics Courses in Bhopal
- Data Analytics Courses in Chennai
- Data Analytics Courses in Jaipur
Quality Control-Use of Data Analytics is of great significance when it comes to Quality control as providing top-notch quality is the main priority for every project manager. Data Analytics could be used to identify key variables that could measure quality and could be used to track any improvement or decline in quality over time and take necessary steps to address any issues related to the decline in quality thereby optimizing the quality control process.
Measuring the Success of the Project-Data analytics provide clear quantifiable answers about the success of the project. Data indicators related to costs, logistics, and different sub-areas could be analyzed furthermore customer satisfaction levels could also be ascertained with the help of data collected from surveys thus giving the project manager a clear idea about the level of success of the project.
Thus, we can say that data analytics is one of the most integral parts of project management as it plays a key role in a whole array of areas ranging from project planning to measuring customer satisfaction. It will become very difficult for project managers to deliver on project milestones without the use of data analytics in project management. The comprehensive application of data analytics makes it an indispensable resource for project managers.
Learn more about:
- Data Analytics vs Data Mining
- Data Analytics Trends
- Data Analytics vs Machine Learning
- Data Analytics Using Power BI
- Data Analytics and Artificial Intelligence
- Data Analytics With Python
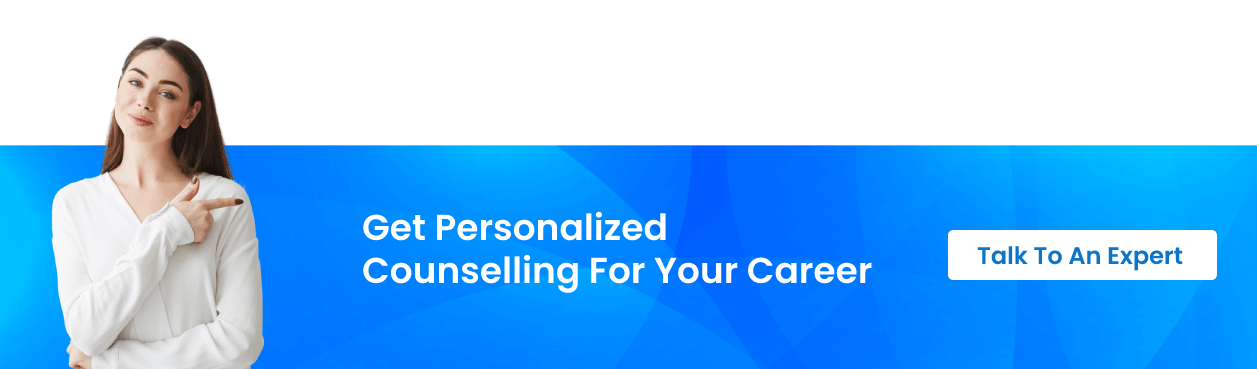
Tools Used for Data Analytics in Project Management
There are a lot of tools of different kinds that are used for data analytics in project management. Such tools simplify the process of data analytics. Some of the most popular tools for data analytics are-
Spreadsheet Software-Spreadsheet software is one of the widely used and easy-to-understand project management. Software such as Google Sheets, Microsoft Excel, Apache OpenOffice, Apple Numbers, and LibreOffice are a few of the popular tools used for data analytics.
Spreadsheet tools help in breaking down data in the form of pivot charts, bar graphs, and pie charts. It also allows the users to apply different kinds of formulas to data so that they can make more sense of the raw data.
Business Intelligence Tools-Business Intelligence tools play a key role in collecting, analyzing, identifying patterns, and visualizing with the help of data. They also provide interactive Dashboards that make it more convenient for the user to understand and use the data. Business intelligence tools also provide comprehensive reports that can give a vital grasp of data.
The tools also allow you to run different kinds of queries for more customized needs. Some of the most popular business intelligence tools are Microsoft Business IB, Tableau, SAP Business Objects, IBM Congo Analytics, and Qilk Sense.
Statistical Analysis Software- These are specialized programs that are meant for users who want to do an in-depth and more complex statistical analysis. They have very powerful libraries which help in dealing with large data sets.
Since they are capable of working on large data sets, they can also be used for financial Modelling and building predictive models. Such tools are used by Business Intelligence Unit departments of various corporations. Some of the most popular statistical analysis software are SAS, Minitab, SPSS, R, and JMP.
Data Visualization Tools-Data visualization tools present data in a visually appealing and in a more interactive manner thus helping the project managers to comprehend complex data easily and making data more usable.
They provide data in interactive visual formats such as tables, pie- charts, Bar Graphs, Histograms, and Infographics. The engaging nature of the data in the form of highly interactive visuals thus helps in better communication and decision-making. Popular data Visualization tools are Tableau, Fusion Charts, QlikView, Highcharts, plotty, and D3js.
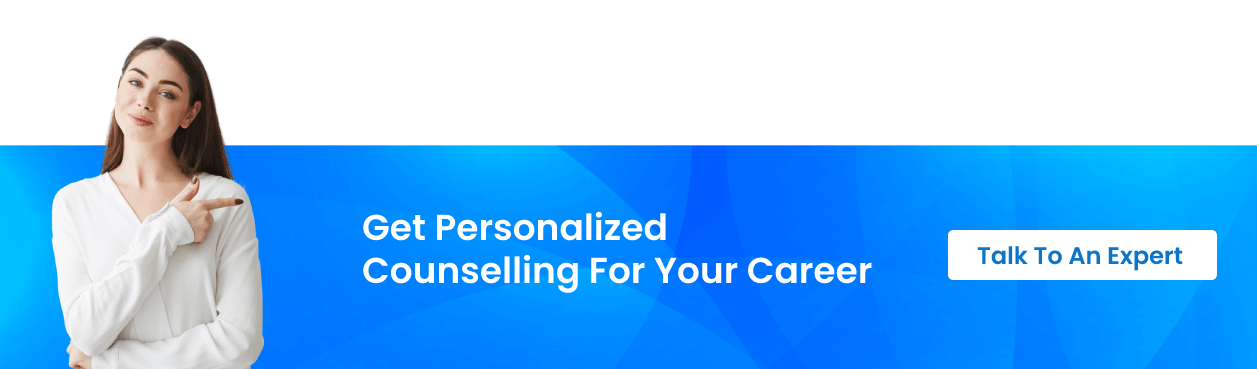
Machine Learning Framework-Machine learning frameworks are highly sophisticated and the most advanced tools of data analytics in project management. Machine learning models are advanced in the sense that the algorithm is trained in a manner that it can identify trends based on past data and build the model.
The model is not static but dynamic since it can reflect and adapt to the changes in data thus making a more precise predictive analysis along with optimizing project schedules. Some of the most popular machine learning frameworks are Py torch, Tensor Flow, Theano, Keras, Scikit learn caffe, and Mx Net.
Text Analytic Tools-Text Analytic tools are a unique kind of application that is used for non-numeric text data. Text Analytic tools are used to make sense of unstructured data. These tools make use of the technique of Natural Language Processing (NLP).
These tools can be used to extract meaningful information from the text data such as identifying the sentiment of the text, i.e. whether the text is written in a positive, negative, or in objective manner in addition to the sentiment analysis these tools can also identify the key highlight or the main theme of the text. Such tools are also capable of extracting vital information from the text such as names or addresses.
These types of tools are mainly used for customer feedback analysis and social media monitoring. Some of the commonly used text analytic tools are Google Cloud Natural, Language API, General Architecture for Text Engineering, -GATE, Datum Box, KH Coder, ODA Miner Lite, and Text Minning extension.
Data Integration and ETL (Extract, Transform, and load) Tools-Data Integration Tools help in extracting data from various different sources and place the combined data in a single place. These tools usually follow a three-step process which consists of extracting the data from different sources, then sanitizing the data and removing irrelevant and superfluous data, and finally loading the sanitized data at the preferred location.
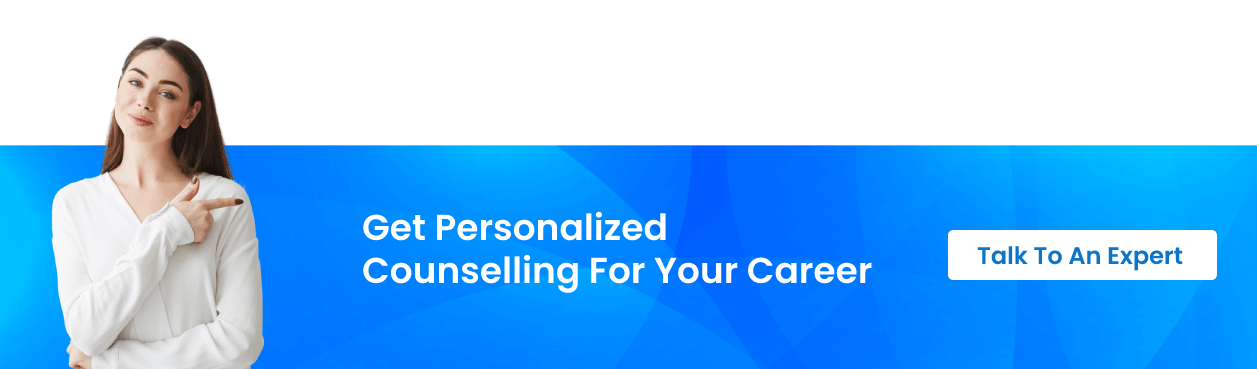
Data integration is a very important part of data analytics since there are vast amounts of data available to any organization but such data is usually scattered over different documents, Data Integration Tools help in getting all the appropriate and workable data in one place thus providing the project managers the right kind of inputs to base their decision making on. Some of the popular Data Integration Tools are Hero Data Matillion, SAS, IBM Infoshare Datastage, Oracle Data Integrator, and Informatica Power Center.
Though, a lot of Data Analytics tools are available in the market for applying data analytics in project management the right tool for any company or user will depend on a variety of factors such scale of the organization, the complexity of data, and the objective of the organization thus depending upon the requirements a project manager can use any single tool or a combination of above-mentioned tools to achieve his goals.
FAQs
How much of a project efficiency could be improved by applying data analytics in project management?
The use of Data analytics in project management can improve project efficiency by many folds but extra care should be taken in choosing the right kind of data analytics tool or a set of tools. Such tools should be chosen which are tailored according to the project.
Data Analytics is performed on past data but the business environment is changing every day so can we completely rely on the use of data analytics in project management to achieve project objectives?
There are various tools such as machine learning tools which not only detect past trends but also make dynamic models that can adapt to changes in data and identify the most subtle trends However, data analytics has some limitations as data analytics tools and their analysis do not take into account extreme events such as natural disasters and political turmoil which can affect the project.
Is it viable for a small business to use data analytics as small data sets are available?
Data analytics is a scientific way of analyzing trends and risks associated with a project it will be equally imperative for a small business to use data analytics in project management to get better at decision making however small business would not need very expensive sophisticated data analytics tools for small business basic data management tool will be enough.