In today’s scenario everyone is competing with each other and in the business world every company wants to get ahead of their rival companies, they want the best and exclusive for themselves. Every company has the desire that their products and services remain best as compared to their rivals. But to achieve their goals, they need research information and data. Precisely to fulfill this need, the concept of data analytics was realized, therefore in this business world the invention of Data Analysis concepts occurred. In this article we will go in-depth into the data analytics concepts and its right understanding and as a beginner, how you can start your career.
Definition of Data Analytics
Most of the time companies collect bundles of data for their knowledge, but if the data is in the raw form, it is meaningless. In that scenario a data, analyst comes in, who will analyze raw data with orthodox meaningful, actionable insights.
Therefore, Data Analytics Concepts is the science of examining raw data to make closure about that information. It includes a series of steps like inspecting, cleansing, converting, and customizing data to extract useful information and support decision-making.
Following is a Further Breakdown of the Process:
Inspecting
Scanning the raw data to point out the initial patterns, characteristics, and numbers of interest.
Cleansing
Filtering the faultiness and irregularities in the data to enhance its quality.
Channelizing the data into a format that can be swiftly analyzed.
Customizing–
Using statistical and computational methods to examine the theory and speculate outcomes.
Data Analytics is pivotal because it helps businesses make decisions, forecast market trends, improve operational quality, and personalize customer participation.
In our day-to-day life also, data analytics plays a crucial role, sometimes without realizing it. For example, before leaving our home, we check the weather forecast to decide what to wear. This forecast is the result of scanning the meteorological data to speculate the weather conditions.
Similarly, when we prepare a cup of tea, we might unknowingly use data analytics by estimating the right proportion of milk to water based on our former experiences to make it a perfect outcome.
These everyday examples reflect Data Analysis, gathering information, analyzing it, and making decisions based on the gained knowledge.
A Must Read,
- Data Analyst Skills
- Data Analytics Course Fee
- Data Analyst Career
- Data Analytics Scope
- Free Data Analytics Courses
- Data Analyst Interview Questions
- Data Analytics Jobs
- Data Analytics and Data Science
- Data Analyst Salary in India
- Data Analyst Course Syllabus
Why Data Analytics Concepts Are Important?
Data Analysis assists businesses to gain more observability and extensive understanding of their product and services. It provides them with a detailed understanding of the customer observation and the customer’s difficulties.
By transferring the pattern beyond data to connect comprehension with the taken measure, companies can design personalized customer experiences, create related digital services, maximize operations, and enhance employee creativity.
Five Key Elements Make Data Analytics Important for Companies:
Gain a Better Understanding of the Target Market-
By capitalizing the consumer information, companies can get a better understanding of buyers’ preference, their needs, and their purchasing trends. This helps companies strengthen their strategies, encourage consumer faith, and boost their sales. If the owners don’t take notice, they risk losing their customer base to a rival who does.
Boost Decision-making Aptitude-
Data analytics helps organizations make fast, wise decisions, minimize costs, and optimize efficiency. It provides proactive opportunity identification based on data rather than assumptions.
However, organizers need to understand that data can show some patterns but a flaw in the analysis could lead to adverse results so it must be used with a critical eye.
Generate Selected Strategies and Marketing Drives:
Data analytics helps organizations create strategies and marketing drives that ensure they reverberate with the selected consumers. By calculating current and transactional statistics, organizations can make customized advertisements, boost marketing value, increase consumers’ beliefs, and faith, and exceed growth.
Refine Operational Inefficacy and Reduce Risk:
Another advantage of data analytics is the skill to enhance operational efficiency. By collecting targeted information and feedback, companies can discover trends that notify products and maximize services.
It also helps to identify areas for streamlining to enhance efficiency and cut the cost. Organizers can use understanding to focus on the most profitable projects. It allows companies to modify their strategies, manage risk, and anticipate future problems.
Recognize New Products and Service Opportunities:
When it comes to new products and services, data analytics allows companies to recognize their present selected consumers, their needs, and trends. It also creates new opportunities to meet their needs. Data analytics concepts can monitor product performance in real time.
Organizations can update their current products by keeping an eye on rival’s actions and plans. Data analytics tailor marketing efforts for greater impacts. It enhances consumer services and fosters loyalty.
By imposing these insights companies can stay graceful, make informed results, and create a competitive environment in the marketplace. Data analytics concepts modify the way organizations manage, create, and grow.
Check Here For More Guidelines On Data Analytics:
- Data Analytics Entry-level Jobs
- Data Analytics Trends
- Data Analytics And Business Intelligence
- Data Analytics Services
- Data Analytics Courses After 12th
Difference Between Data Analytics Concepts and Data Science
Many of us might be confused with Data Analytics Concepts and data science and might consider them to be the same career. But they are very distinct fields. Beginners need to understand that both have different impacts on businesses.
Data Analytics Concepts focuses on refining and conducting computational analysis on existing datasets. Whereas, data Science integrates the scientific method, math, arithmetic, and specialized programming. Despite their differences, it is crucial to understand that data science and data analytics both work together.
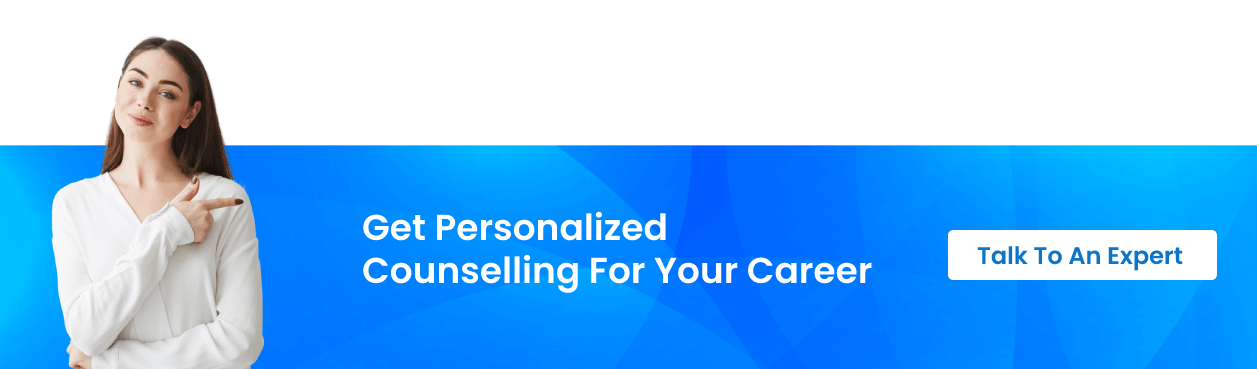
Their Approach to Data:
Data Analysts focus on illuminating present data to get results from existing questions. They use systematic applications to form visual representations like charts and graphs that help organizations make choices based on present data.
However, Data Scientists dig in deeper. They not only get solutions from present queries but also innovate new queries for the future. Their work demands constant improvement of these models as new information comes in.
Their Application and Software:
Data analysts are expected to excel in programming languages like R, SQL, SAS, and Python. Data scientists are expected to excel in Hadoop, Java, and Python applications.
Recommend Read,
- Google Data Analytics Certification
- Data Analytics Books
- Data Analyst Course Eligibility
- Data Analytics Qualifications
- Data Analytics For Business
Different Types of Data Analysis
Now, this is the main part of Data Analytics Concepts. Data analysts can convert raw information into fruitful facts and figures by calculating inclinations, alterations, and patterns. This whole process helps to take future outcomes in the organization and resolve the current problems that are creating hurdles in the revenues and other business exercises.
Let’s take a look at the four main types of Data analysis: descriptive, diagnostic, predictive, and prescriptive analysis.
1. Descriptive Analysis
It is like taking a picture of previous data and representing it in a layman format. It is the exercise of assembling and summarizing the previous facts without making assumptions. It is about “what occurred” in history. The two main tools used in this analysis are Data Accumulation and Knowledge Discovery in Data.
Data Accumulation–
It is the collection and assembling of the information into abstract form.
Knowledge Discovery in Data–
It is the transfer of abstract information to discover patterns.
The main objective is to show the data only through graphs or charts so that they can be understood by a large audience.
2. Diagnostic Analysis
It is like being an investigator with information. Diagnostic analysis is about why it occurred. Let’s dig into how it works:
Identify the odd one out – Firstly, identify any unusual thing in data, like a sudden drop in sales.
Uncovering phase- The next step is, to collect additional data sources that might explain why such anomalies arose.
Uncover casual relationships– Now, look for connections that could explain oddities, like episodes that correspond with the decline in sales.
Utilize the Methods- Finally, data analysts use methods like probability, regression, and time series analysis to confirm these connections.
So, this analysis helps us acknowledge the root cause behind the information trends.
Looking for other best options? Dig in here:
- Data Analytics Courses in Mumbai
- Data Analytics Courses in Delhi
- Data Analytics Courses in Kolkata
- Data Analytics Courses in Bangalore
- Data Analytics Courses in Pune
- Data Analytics Courses in Gurgaon
3. Predictive Analysis
This analysis tries to determine what is likely to occur in the future. Data Analysts use previous data and math to make reasonable predictions. Data analysts take the following steps for predictive analysis:
Archival Record- Take a look at what occurred before.
Possibility- Uses arithmetic skills to find out the possibility of something happening again.
Predictions- Makes forecasts like which products will sell or how sales will incline or decline.
Hence, predictive analysis helps organizations plan better and execute smarter choices.
4. Prescriptive Analysis
It is like a strategic advisor for an organization. Data analysts use the predictions from predictive analytics and recommend the best action to take. Here’s a simple breakdown:
Situation Analysis- It glances at different future situations based on the data.
Decision Making- It helps organizations to figure out what to do to make the most of the predicted results.
Intricate Skills – It uses skills like algorithms and machine learning to give smart advice.
Major Influence- The effective use of prescriptive analytics can have a major influence on a business’s decision-making and profits.
It is also crucial to match the analysis with the correct data type- whether it is nominal, ordinal, intervals, or ratios. Each type of data can tell you different requirements.
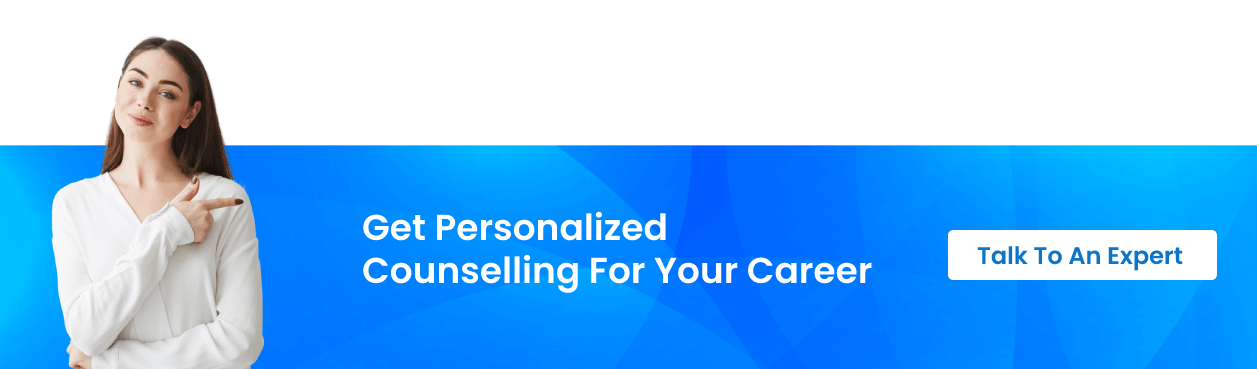
What is Data Types?
For Data Analytics Concepts, the comprehension of Data types is very important. A data type is a fragment of data that commands a computer system how to explain its value. Data types interpretation makes clear that pieces of information are collected in the selected pattern and the value of each property is expected.
Following are the precise descriptions of the different data types: Quantitative and Qualitative Data.
1. Quantitative Data
It is also referred to as numerical data. The information here is in numbers and can be calculated. For example, our age, temperature, and data about income, all come under quantitative data. There are two types of numerical data: discrete data and continuous data.
Discrete Data
Information that is countable and can not be split into smaller pieces of information. It consists of definite and discrete values.
For example number of two-wheelers in a parking lot, the number of female employees in a corporate office, and the number of tickets sold in Cineplex.
Continuous Data
It represents a variation of values. In this data type information can be divided into smaller parts. It can take
any value within a confined scale.
For example, the height, weight of students in the class, and temperature were recorded in March month.
2. Qualitative Data
Data that can not be calculated in numerical is called qualitative data. It is also referred to as Categorical Data. This data can be gathered in the form of pictures, signs, sound clips or messages and responses.
Qualitative data enhances factors to our research, to investigate more on predictions and our point of view.
This can be further divided into two types: Nominal Data and Ordinal Data.
Nominal Data
This data has no number order. It represents categories without any innate order.
For example, data on the gender of the student, raw information on the favorite color of people, and the most favorable language of employees.
Ordinal Data
This type of data falls into the most specific order. It has some kind of numeric presence. Ordinal data lacks even breaks. The variation between sets may not be similar.
For example, customer reviews can be uneven and a company survey ratings may not be the same as the gap between happy and unhappy.
You should check the courses in other rewarding cities
- Data Analytics Courses in Hyderabad
- Data Analytics Courses in Noida
- Data Analytics Courses in Ahmedabad
- Data Analytics Courses in Agra
- Data Analytics Courses in Chennai
- Data Analytics Courses in Chandigarh
What is Data Collection?
Data collection is a methodical procedure of collecting pieces of information and measurements. Whether you are conducting a survey or analysis for a corporation or government organization, data collection gives you an enhanced and original understanding of your survey queries.
Following Are the Important Steps of Doing Data Collection:
1. Interpret the Target of Your Survey:
- Before gathering information, recognition of your target is very important. Initiate the survey by outlining the problematic factors which you want to convey in your research.
- Compose all your survey queries that interpret what you want to know.
- Based on your survey questions, you might need to gather information either on numerical data or categorical data.
2. Select your Data Collection Method
Depending on the types of information you want to gather, the following are the most reliable methods of data collection.
- Polls- It is a method to gather information from a sample of the people and count as a survey of the whole population. It consists of some questionnaires.
- Interview- Dialogues with the audience or person to collect data. It is a one-to-one conversation.
- Monitoring – Organized monitoring of episodes and actions.
- Practical- Systematical conduct to examine the issue and its cause.
- Secondary data analysis- Using present information from other origins.
3. Programme Your Strategy
Strategize a detailed course of action for data collection. There are the following steps for planning the procedures.
- Sampling- It is a technique of collecting specimens or instances or participants.
- Information Source- Where you will gather your information, for example, database, fieldwork.
- Data document- How you will record reactions and opinions.
- Ethical Consideration- Reassure audience consent and privacy.
4. Gathered Your Information
- Carry out your course of action by collecting data according to the selected method.
- Assure the data quality, perfection, and connection to your survey objectives.
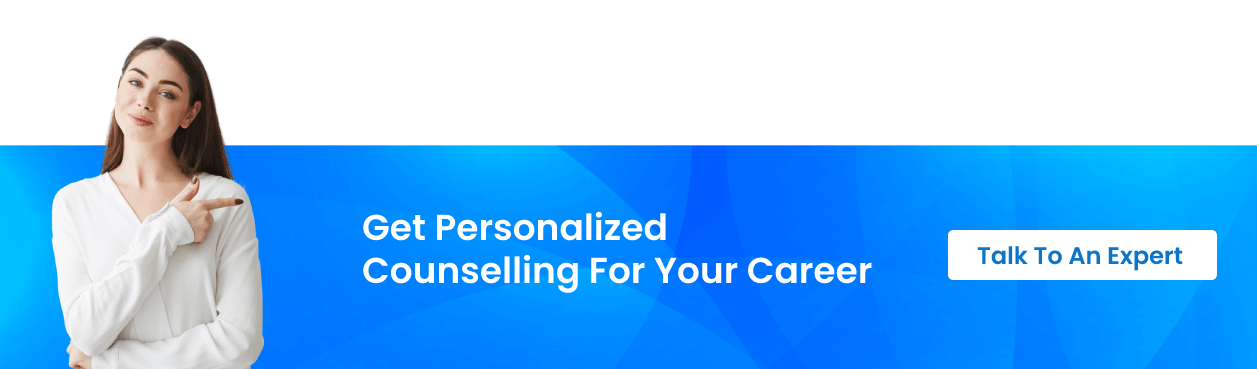
What is Data Cleaning?
It is the main important step of Data Analytics Concepts, it is also known as data cleansing. It is the process of composing data for examination by picking and correcting mistakes, inaccuracies, and unpredictability within a data set.
It involves removing variations and assuring that the information is ready for the right examination.
Hereby Are the Tasks Included in Data Cleaning:
Eliminating Identicals- Recognizing and removing duplicate data or records. Duplication of information occurs when it is gathered from many sources, many data sets are used, and when removing data. This affects the productive data analysis calculation procedure.
Unimportant data is another subject that has to be considered. This whole procedure of cleansing makes the ultimate data more accessible and applicable to the primary objective and manufactures the least distraction from the primary goal.
Dealing with lost values- Handling the insufficient or negligible values. Missing rows and columns of information affect the correct data analysis procedure sometimes it also leads to failing results.
It can be monitored and then removed from the primary data source or can be replaced by the predictions
Systematizing formats – Ensuring regular representations like date, units, and formats.
Rectifying faultiness- Usually, data is collected in three types, boolean, numerics, and strings. Sometimes numbers can be written in text instead of digits, there can be typing mistakes and spelling mistakes. These all need to be rectified and put in the correct format.
Demanded Expertise Required for a Data Analyst
Up to this point, we have concisely talked about the types of data analysis and its significance. Now, let’s investigate the crucial essentiality of a data analyst. We will study all the essential expertise and classification that one should own.
1. Technical skills
- Numerical and Demographical Ability- Data analysts spend most of their time working with numerals, therefore you have to be good with numerics.
- Software Knowledge- Also, data analysts have to rely on software languages such as Python, SQL, or R to carry out their work. This may seem difficult at first, but you can learn it over time.
- Logical Mindset- A data analyst must interpret data. It’s not enough to just rely on numbers and share your findings. A data analyst needs to be able to understand what’s going on and to study deeper if necessary. So, a logical perspective is a must.
- Information Visualization- The skill to present complex information, visually using tools like histograms and pie charts, is key to communicating effectively. There is no point in doing all the study if an analyst doesn’t have an effective way to put those understandings together and interface them to investors.
2. People Skills
- Critical Thinking- Analysts must skillfully choose from a variety of tools and techniques to solve different challenges. Data analytics is all about solving business problems and that requires some acute critical thinking skills.
- Social Intelligence- Once you have gathered data from your understanding, it’s crucial to share your results in a way that gives profit to a business.
A data analyst works in close association with business investors and may be accountable for sharing and presenting to the whole company. So, if you are thinking of pursuing Data Analysis, it is mandatory to have excellent social intelligence for your job.
- Flexibility- The field of Data Analytics Concepts is changing constantly. This means that it is important to keep a broad mind and be aware of new methods and technologies. Try to make your understanding a key part of how you work- this will give you an advantage in this field.
These are all expertise and qualifications that are elementary for a data analyst to successfully provide the best outcome to an organization.
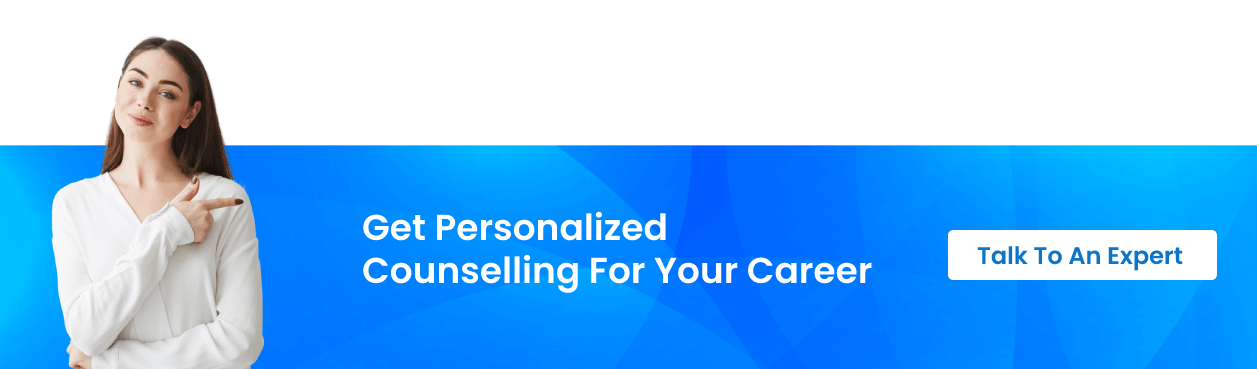
FAQs
Q. Which data analytics has the most value?
Prescriptive Data analytics is the most up-to-date form of data analysis because it advocates the optimal course of action.
Q. Can AI replace a data analyst’s post?
AI can’t replace the data analyst job, but AI will help an analyst in handling projects.
Q. Does Data analytics need coding skills?
A data analyst is expected to be skilled in coding languages.
Q. Can a non-mathematical candidate apply for a Data Analytics course?
Any graduate undergraduate and all professionals can apply for this course.
Conclusion
The above mentioned are all the information and knowledge of the fascinating field of Data analytics concepts. In this article, we tried to give you an extensive understanding of data analysis which includes types of analysis, tools of data, description of data science, and from where you can gain the knowledge of data analytics.
If you want to pursue your career as a data analyst you will need to become more proficient in all the skills, tools, and techniques.
In the present situation, data analytics is among the top fields of research and investment purposes. New forms of content-driving analysis and artificial intelligence will replace the present technologies. Therefore to grasp the power of new techniques organizations need to know all about data analytics which makes the companies drive business success.